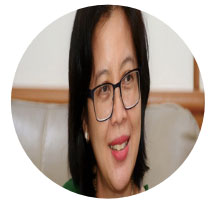
Dr. Selvia Vivi Devianti,
Head of Research and Development Directorate, BPK
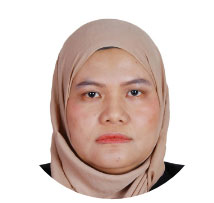
Ms. Dwi Amalia Sari,
Directorate General of Audit IV, BPK
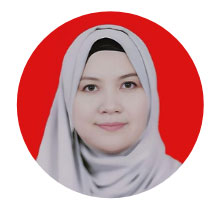
Ms. Yenni Elvariza,
Directorate General Of Investigate, BPK
Abstract
Conducting assurance service on sustainability reports is crucial to support endeavours in climate change mitigation, yet, many find such an audit challenging. SAI Indonesia considered it is important to have audit guidelines to help SAI auditors perform the assurance of sustainability reports effectively. This paper argues the possibility of implementing technology in conducting assurance for SR and SAIs could embrace AI and Machine Learning (AML) as tools for implementing the guidelines. ChatGPT, Blackbox.ai, and Google Colab are utilised for this experiment.
The result suggests that AML are useful for auditing sustainability reports. ChatGPT and Blackbox.ai help analyse the documents and structure the report quite satisfactorily, while Google Colab provides insights and red flags. Yet, before integrating AML into the implementation, SAI should address issues such as assuring data safety and tailoring the AML algorithm to auditing contexts.
We recommend that SAIs should design and develop their own AML for more optimised outcomes.
Keywords: AI; Sustainability Report; Assurance; Audit.
Introduction
Sustainability reports provide valuable information on how companies address social, environmental, and financial aspects of their business (Chopra et.al., 2024). However, the non-financial report is voluntary, and companies are allowed to choose the reporting standards. Hence, much information in the reports, such as ethical practices and community engagement, is prone to biased presentation (Hahn & Kühnen 2013).
Assurance in sustainability reporting, therefore is essential. Assurance provides more credibility to the company’s report (Kwarto.et al., 2024). Public banks increasingly reward companies that demonstrate a genuine commitment to sustainable practices (Wagenhofer, 2024). Assurance service encourages companies to improve the quality of sustainability reports (Blessing, 2024).
Yet, auditing sustainability reports is challenging. Unlike the Financial Statements, sustainability reporting lacks universally accepted frameworks (Moodaley & Telukdarie (2023). Standards use different metrics, disclosures, and formats, which complicate the assessment (Pizzi. et al., 2024). Also, claims over qualitative information are hard to assess, especially when proxy indicators have multi-interpretation (Yusuf et al., 2024). Sustainability data often involve suppliers and third-party providers which sometimes are unverifiable (Walker. et al, 2024).
Anticipating the pronouncement of the IPSASB Sustainability Reporting Standard Exposure Draft and IAASB International Standard of Sustainability Reporting Assurance 5000, SAI Indonesia has started an analysis project. The project emphasises that SAI should prepare a Sustainability Report and conduct Assurance to Sustainability Reports. Four steps in the sustainability reports assurance are 1) Planning and Scoping; 2) Evidence Gathering; 3) Evaluation; and 4) Reporting (INTOSAI WGEA. 2023).
In this paper, we attempt to utilise AML in the audit process to improve the quality of the assurance services.
AML Utilisation in Auditing
Using AML for conducting assurance on sustainability reports offers some advantages. AML can analyse massive data accurately, and automate data extraction and aggregation continuously (Jejenjiwa, 2024). It also helps auditors cross-reference data, analyse text and sentiment with external sources on the internet ( Thottoli, 2024). Moreover, AML can perform predictive analysis to assess whether a company is on track to meet future sustainability goals (De Villiers, et al. 2024). During reporting, AML can compare different sustainability reports and verify sustainability claims (Petcu, et al. 2024).
Yet, AML also has major pitfalls to consider. AML can produce false results due to inaccurate data (Almaqtari, 2024). AML is also prone to misinterpreting context due to a lack of humane judgment. (Robert, 2024). Most importantly, the use of AML raises data privacy concerns and ethical questions about accountability (Laine, 2024; Sachan, 2024). These issues need to be solved before adopting this new audit tool.
This study highlights the importance of combining AML with human expertise and judgment. Effective AML integration requires ongoing monitoring, transparency, and careful alignment with ethical standards and regulatory requirements (Ojewale, 2024).
Research Methodology
In this study, we experiment with 2 open-source AI and 1 machine learning. We intentionally use different organisations so that the open-source AI does not pick up the pattern, withdraw misleading conclusions, and share false deductions with the public. We also used online documents. The organisation names are hidden, for privacy reasons.
We used chat GPT 4.0 for the Planning and Scoping. We uploaded ISAE 3000 and the Sustainability Report of c ompany X(name retracted), an oil and gas company. Chat GPT was requested to define the scope and objective of the assurance engagement. We created two different prompts asking the same questions. The first prompt directly asked the question. The second prompt uses roleplay before asking the same question.
For the second step, evidence gathering, we utilised Google Colab to analyse sentiments. Company Y (name retracted) operates in the pulp paper business. In its sustainability report company Y claimed that it has a highly regarded reputation for its contribution to society. We tested this claim with sentiment analysis using available data on the internet.
For the third step, Evaluation; we evaluated the practice of sustainability reports. Company Z (name retracted), a multinational consumer goods company, claimed it has strong commitment towards society. We analysed its CSR spending over a period of time, and compared it with the profits to obtain the percentage allocated to the sustainability endeavours. Using Google Colab and Machine Learning, we used the data to conduct a prediction of the likely CSR spending in the future.
For the fourth step, Reporting we used Blackbox.ai to write an assurance report for BPK’s 2020 Sustainability Report. The report has been audited with an unqualified opinion. We asked Blackbox.ai to act as an external auditor conducting assurance service to BPK’s report using GRI as the sustainability standard and ISAE 3000 as its assurance standard.
Findings
We found that Chat GPT provides quite useful insights, albeit the limited data uploaded to the AI. Company X had some records of environmental violations. Yet, recently Company X once good reputation has started to retaliate. The company emphasises its endeavour toward more sustainable business practices in the reports, hence, we tested if Chat GPT considers this background in its answers on the scope and the objective of the audit. The answer of Chat GPT is as below
The responses were actually quite justified, despite the fact that they are not specifically addressed to Company X and are applicable to many other organisations as well. Nevertheless, the responses were useful for auditors to initiate further analysis and help structure the audit process.
For the second step; Evidence Gathering, our finding on sentiment analysis about company Y has shown interesting results. Regardless of the company’s claim in its Sustainability Reports, Company Y has more negative sentiments from the public on the internet for the periods claimed.
For the third step, Evaluation, Company Z’s commitment to sustainability is reflected by the increasing CSR funding over periods of time. The streamlined graph shows that company Z has indeed allocated an increasing amount of its profit for CSR. However, our prediction of the future trend suggests that after some periods of time, the CSR spending from company Z will decrease significantly.
For the final step: Reporting, Blackbox.ai’s response was similar to the actual assurance. Unlike ChatGPT, the roleplay prompt in Blackbox.ai was unnecessary. Using the role-play prompt, Blackbox.ai fabricated imaginary data and information. The AI, for example, stated in the report that the assurance engagement included interviews and field observations. We therefore used prompts without role-play scenarios.
The AI responses to the prompt were as below.
The assurance report generated by Blackbox.ai was relatively concise and accommodated all the necessary elements quite comprehensively. The AI-generated report was also structured and can be improved by adding necessary information as depicted (Exhibit a).
Conclusion
The application of AML in the assurance of sustainability reports has demonstrated significant potential. Auditing sustainability reports poses unique challenges due to its non-financial information and non-compulsory implementation. AI-assisted audits offer deeper analysis and insight.
Our experiments with different AML for conducting assurance on sustainability reports showed some mixed results. ChatGPT tends to produce better answers if the prompt accommodates role-playing. In contrast, Blackbox.ai works better with straightforward instructions. Both open-sourced AI models, however, are not designated for auditing tasks, hence, most jargons used in the answers are not audit-specific. Conversely, Google Colab in the evidence gathering and evaluation phase was quite useful in providing insights and possible red flags. Yet, none of these tools are reliable enough to withdraw conclusions for audit finding.
Considering the potential, we support the use of AML in auditing, especially for auditing non-financial data and information. However, for more effective results, we suggest that SAIs should allocate adequate resources for developing a designated AI specifically for the tasks.
Further studies therefore should aim at tailoring AI into proper auditing tools.
Exhibit a
Independent Assurance Report on BPK’s 2020 Sustainability Report
To The Management of Badan Pemeriksa Keuanan (BPK)
Introduction
We have been engaged to perform a limited assurance engagement on the 2020 Sustainability Report of Badan Pemeriksa Keuangan (BPK) in accordance with the International Standard on Assurance Engagements (ISAE) 3000. The objective of our engagement is to provide assurance on whether the report is prepared in accordance with the Global Reporting Initiative (GRI) Standards.
Management's Responsibility
The management of BPK is responsible for the preparation of the Sustainability Report in accordance with the GRI Standards. This responsibility includes establishing and maintaining internal controls relevant to the preparation of the report that is free from material misstatement, whether due to fraud or error.
Our Responsibility
Our responsibility is to express a conclusion on the Sustainability Report based on our assurance engagement. We conducted our engagement in accordance with ISAE 3000, which requires us to comply with ethical requirements and plan and perform the engagement to obtain limited assurance about whether the report is free from material misstatement.
Scope of the Engagement
The scope of our engagement included:
1. Reviewing the BPK Sustainability Report against the GRI Standards, specifically focusing on GRI 102 (General Disclosures), GRI 200 (Economic Topics), GRI 300 (Environmental Topics), and GRI 400 (Social Topics).
2. Evaluating the processes and controls in place for data collection and reporting.
3. Assessing the completeness and accuracy of the reported information.
Methodology
Our procedures included:
1. Inquiries of management and relevant staff responsible for the preparation of the Sustainability Report.
2. Review of the report against the GRI Standards.
3. Examination of supporting documentation and data sources.
4. Analysis of the data collection and reporting processes.
Conclusion
Based on our limited assurance procedures, we conclude that nothing has come to our attention that causes us to believe that the BPK Sustainability Report for 2020 is not prepared, in all material respects, in accordance with the GRI Standards.
Key Observations:
1. General Disclosures (GRI 102): The report adequately includes relevant organizational information, governance structure, and stakeholder engagement processes.
2. Economic Topics (GRI 200): The report provides sufficient information regarding economic performance and anti-corruption measures.
3. Environmental Topics (GRI 300): The report discusses environmental impacts, but further detail on specific metrics such as emissions and energy usage would enhance compliance.
4. Social Topics (GRI 400): Social impacts, including employee welfare and community engagement, are well-documented.
Recommendations for Improvement:
1. Enhance Environmental Metrics: Include specific targets and comprehensive metrics related to environmental performance.
2. Strengthen Data Collection Processes: Improve documentation supporting data collection to ensure transparency and reliability.
3. Expand Stakeholder Engagement: Increase the depth of stakeholder feedback and responses in future reports.
References
Abdullah, A. A. H., & Almaqtari, F. A. (2024). The impact of artificial intelligence and Industry 4.0 on transforming accounting and auditing practices. Journal of Open Innovation: Technology, Market, and Complexity, 10(1), 100218.
Almaqtari, F. A. (2024). The Role of IT Governance in the Integration of AI in Accounting and Auditing Operations. Economies, 12(8), 199.
Blessing, M. (2024). The Role of IFRS in Promoting Sustainability Reporting: Aligning with Global ESG Standards.
Chopra, S. S., Senadheera, S. S., Dissanayake, P. D., Withana, P. A., Chib, R., Rhee,
J. H., & Ok, Y. S. (2024). Navigating the Challenges of Environmental, Social, and Governance (ESG) Reporting: The Path to Broader Sustainable Development. Sustainability, 16(2), 606.
De Villiers, C., Dimes, R., & Molinari, M. (2024). How will AI text generation and processing impact sustainability reporting? Critical analysis, a conceptual framework and avenues for future research. Sustainability Accounting, Management and Policy Journal, 15(1), 96-118.
Hahn, R., & Kühnen, M. (2013). Determinants of sustainability reporting: A review of results, trends, theory, and opportunities in an expanding field of research. Journal of cleaner production, 59, 5-21.
INTOSAI WGEA (2023). Sustainability reporting in public sector and the role of SAIs. INTOSAI WGEA 2023-2025.
Jejeniwa, T. O., Mhlongo, N. Z., & Jejeniwa, T. O. (2024). A comprehensive review of the impact of artificial intelligence on modern accounting practices and financial reporting. Computer Science & IT Research Journal, 5(4), 1031-1047.
Kwarto, F., Nurafiah, N., Suharman, H., & Dahlan, M. (2024). The potential bias for sustainability reporting of global upstream oil and gas companies: a systematic literature review of the evidence. Management Review Quarterly, 74(1), 35-64.
Laine, J., Minkkinen, M., & Mäntymäki, M. (2024). Ethics-based AI auditing: A systematic literature review on conceptualizations of ethical principles and knowledge contributions to stakeholders. Information & Management, 103969. Moodaley, W., & Telukdarie, A. (2023). Greenwashing, sustainability reporting, and artificial intelligence: A systematic literature review. Sustainability, 15(2),1481.
Ojewale, V., Steed, R., Vecchione, B., Birhane, A., & Raji, I. D. (2024). Towards AI Accountability Infrastructure: Gaps and Opportunities in AI Audit Tooling. arXiv preprint arXiv:2402.17861.
Petcu, M. A., Sobolevschi-David, M. I., & Curea, S. C. (2024). Integrating digital technologies in sustainability accounting and reporting: Perceptions of professional cloud computing users. Electronics, 13(14), 2684.
Pizzi, S., Venturelli, A., & Caputo, F. (2024). Restoring trust in sustainability reporting: the enabling role of the external assurance. Current Opinion in Environmental Sustainability, 68, 101437.
Roberts, H., Hine, E., Taddeo, M., & Floridi, L. (2024). Global AI governance: barriers and pathways forward. International Affairs, 100(3), 1275-1286.
Sachan, S., & Liu, X. (2024). Blockchain-based auditing of legal decisions supported by explainable AI and generative AI tools. Engineering Applications of Artificial Intelligence, 129, 107666.
Thottoli, M. M. (2024). Leveraging information communication technology (ICT) and artificial intelligence (AI) to enhance auditing practices. Accounting Research Journal, 37(2), 134-150.
Wagenhofer, A. (2024). Sustainability reporting: a financial reporting perspective.
Accounting in Europe, 21(1), 1-13.
Yusuf, M., Dasawaty, E., Esra, M., Apriwenni, P., Meiden, C., & Fahlevi, M. (2024). Integrated reporting, corporate governance, and financial sustainability in Islamic banking. Uncertain Supply Chain Management, 12(1), 273-290.