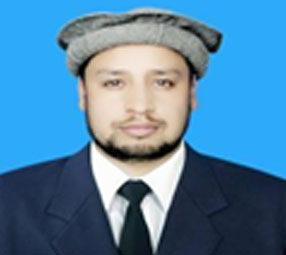
Atta Ur Rehman, Assistant Audit Officer,
Introduction
Artificial intelligence (AI) refers to the ability of digital computers or robot systems to perform tasks typically requiring human intelligence. This includes capabilities such as reasoning, learning from past experiences, discovering patterns and making informed decisions. As AI continues to evolve at a rapid pace, its impact has been transformative, revolutionising industries across the globe. From automating routine tasks to boosting productivity and enabling innovative forms of human-computer collaboration, AI is reshaping sectors like auditing, finance and accounting in ways that were once unimaginable.
However, the widespread adoption of AI brings with it both opportunities and challenges. While AI has the potential to drive efficiency and innovation, it also raises concerns about job displacement, increased reliance on technology and the risk of bias in decision-making due to flawed algorithms.
In Pakistan, the government is actively pursuing the integration of AI into the public sector with the goal of improving governance, service delivery and socioeconomic development. A range of initiatives and strategic frameworks have been put in place to guide this process, despite the challenges the country faces in terms of infrastructure and resources.
One of the key steps in this direction is the introduction of the National AI Draft Policy, which sets out a comprehensive strategy for AI adoption in public services. The policy emphasizes the importance of fostering public-private partnerships, aligning educational programs with emerging technological trends and advancing e-governance initiatives across critical sectors such as healthcare, education and commerce.
Under the Vision 2025 framework, Pakistan has placed a strong emphasis on digital transformation in governance. This includes upgrading IT infrastructure, implementing e-governance strategies to improve service delivery and enhancing citizen engagement. The vision also outlines plans to establish specialised institutes dedicated to AI research and development, ensuring the country remains at the forefront of technological innovation.
To further accelerate AI adoption, the government is encouraging collaboration between public institutions and the private sector. By leveraging the expertise and resources of private companies, Pakistan aims to develop AI applications that address the unique challenges of the public sector while driving sustainable innovation.
In parallel, the Higher Education Commission (HEC) has approved numerous universities to offer AI- related programs, empowering the workforce with critical skills for the digital age. HEC has also established key research centres, such as the National Center for Artificial Intelligence (NCAI) and the Sino-Pak Center for Artificial Intelligence (SPCAI), which are working to push the boundaries of AI research and development.
Moreover, efforts are being made to enhance IT infrastructure in underserved regions, ensuring equitable access to AI-driven services. This includes the creation of technology zones and software parks designed to nurture technological growth and promote digital inclusion across the country.
Opportunities of AI and Machine Learning in Public Sector Auditing
1. Improve Accuracy
AI tools can process and analyse large datasets with high precision, reducing the chances of human error and enhancing the reliability of audit results. The integration of AI tools into auditing processes significantly boosts precision, reduces human error, and enhances the reliability of audit results. By processing large datasets with advanced algorithms and maintaining objectivity in analysis, AI transforms how audits are conducted. This leads to more accurate assessments, timely identification of issues, and ultimately a stronger foundation for accountability in public sector entities. As organisations continue to embrace these technologies, the future of auditing looks increasingly promising and efficient.
Human auditors are susceptible to biases and subjective judgments, which can lead to inconsistencies in audit results. AI systems operate on objective data analysis, significantly reducing the risk of human error. This objectivity ensures that audits are based on factual evidence rather than personal interpretations.
AI tools apply consistent criteria across all data sets. Unlike human auditors who might vary their approach based on experience or intuition, AI ensures that every dataset is evaluated against the same standards. This uniformity enhances the reliability of audit results.
AI and ML can enhance the accuracy of audit processes by minimising human error. Automated systems can process large volumes of data consistently and reliably, leading to more precise outcomes in the audit findings.
2. Enhance Efficiency
AI and Machine Learning (ML) can automate repetitive tasks, allowing auditors to focus on more complex analyses. This can lead to significant time savings and improved accuracy in audit processes. Many tasks in auditing, such as data entry, basic calculations, and compliance checks, are repetitive and time-consuming. AI and ML can take over these tasks, performing them faster and often with fewer errors than humans. This transformation not only saves time but also enhances the quality of audits, allowing auditors to concentrate on higher-value tasks that require their expertise. Overall, this leads to a more effective and efficient audit process.
While not exclusively mentioned, Robotic Process Automation (RPA) technologies are likely being considered for automating routine tasks such as data entry and report generation, thus freeing up auditors to focus on more complex analytical tasks.
RPA focuses on complex analyses by automating routine tasks, auditors can dedicate more time to complex analyses that require critical thinking, judgment, and expertise. This might include evaluating financial trends, assessing risk factors or interpreting unusual transactions. Automating repetitive tasks significantly reduces the time auditors spend on mundane activities. This efficiency allows them to complete audits more quickly and take on more clients or projects.
3. Better Data Analytics
These technologies enable the analysis of large datasets, which can uncover patterns and anomalies that human auditors might miss. For example, AI tools have been utilized by Supreme Audit Institutions (SAIs) to detect high-risk contracts in public procurement.
AI and ML technologies play a crucial role in analys ing large datasets, enabling the discovery of patterns and anomalies that human auditors might overlook. This capability is particularly valuable in auditing contexts, such as public procurement.
AI and ML can process vast amounts of data efficiently. This ability allows data to be analyzed from various sources simultaneously, leading to a more comprehensive Breakdown of the Paragraph.
One of the strengths of AI and ML is their capacity to identify subtle patterns or irregularities within the data that may not be apparent to human auditors. These technologies utilize sophisticated algorithms to sift through large datasets, detecting trends or anomalies that could indicate potential risks or fraudulent activities. For instance, they can flag unusual spending behaviours or highlight contracts that deviate from established norms.
4. Efficiently Detection of Fraud
AI systems utilise machine learning algorithms that are trained on historical transaction data. This training allows the AI to understand what constitutes normal behaviour within specific datasets. By establishing a baseline of typical activities, these systems can effectively identify deviations from this norm, which may indicate fraudulent behaviour. For example, if an organisation typically processes transactions of a certain size, any transaction that significantly exceeds this size can be flagged for further investigation.
By integrating Pattern Recognition and Real-Time Monitoring techniques, AI systems make it significantly easier for auditors within SAIs to identify suspicious activities. The ability to process large datasets with high precision reduces the chances of human error, which is often prevalent in traditional auditing methods. As a result, auditors can focus their efforts on investigating flagged anomalies rather than sifting through vast amounts of data manually.
The proactive nature of AI-driven fraud detection means that potentially fraudulent activities can be identified and addressed before they escalate into significant issues. This not only enhances the reliability of audit results but also strengthens public trust in the auditing process by demonstrating a commitment to transparency and accountability.
The use of AI systems within Supreme Audit Institutions leverages historical data and advanced anomaly detection techniques to improve fraud detection capabilities. This leads to more accurate identification of suspicious activities, ultimately helping to prevent fraud and enhance the integrity of public audits.
5. Improvement in Decision-Making Process
AI can assist in making informed decisions by providing insights derived from data analysis. For instance, it can help identify compliance risks and fraud, thereby enhancing the overall governance framework within public institutions.
AI enhances decision-making by analysing large volumes of data and extracting valuable insights. This means that decisions can be based on evidence and trends rather than intuition alone.
Through advanced algorithms and machine learning techniques, AI can process complex datasets to reveal patterns, anomalies and correlations that may not be immediately apparent to human analysts.
One specific application mentioned is the identification of compliance risks (potential violations of laws or regulations) and fraud (deceptive practices). By detecting these issues early, AI helps organisations mitigate risks before they escalate.
The overall governance framework refers to the systems, processes and policies that guide an organisation’s operations. By integrating AI into this framework, public institutions can improve transparency, accountability and efficiency in their operations.
6. Development in Personalize Services
AI-driven systems are increasingly being integrated into public services to enhance citizen engagement and satisfaction through personalised services. This approach is particularly relevant in the context of public auditing, where tailored services can significantly improve interactions between citizens and government entities.
AI tools can facilitate better communication between public auditors and citizens. By utilising natural language processing (NLP), AI can interpret citizen inquiries and provide immediate, relevant responses. This responsiveness helps demystify the auditing process and encourages greater participation from the public, as they feel their concerns are being addressed effectively.
With AI-driven insights, public auditors can identify patterns in citizen behaviour and preferences. This allows for the design of services that are more aligned with community needs. For example, if data shows that a specific demographic frequently accesses particular services, auditors can ensure those services are readily available and easy to navigate.
Personalised services foster a sense of transparency in public audits. When citizens receive tailored updates about audit processes or outcomes that directly affect them, it builds trust in the system. Auditors can use AI to provide real-time updates or insights into how audit findings may impact specific communities or groups.
AI systems can aggregate and analyse data from various sources to inform public auditors about potential areas of concern or interest within the community. This proactive approach enables auditors to focus on high-risk areas or issues that require immediate attention, ultimately enhancing the effectiveness of audits.
7. Cause of Cost Reduction
The integration of AI and ML into Supreme Audit Institutions (SAIs) has the potential to significantly reduce operational costs while enhancing the efficiency and effectiveness of public sector audits.
These technologies lead to significant cost savings by reducing the need for extensive manpower and minimizing the duration of audit cycles. For instance, electronic auditing tools can streamline processes and reduce the costs associated with physical audits.
8. Predictive Analytics
The integration of AI and ML in public audit institutions significantly enhances their ability to forecast potential risks and compliance issues. This capability arises from the analysis of historical data and current trends, enabling organisations to proactively address problems before they escalate.
Predictive analytics involves using statistical techniques, machine learning algorithms, and data mining to analyse historical data and predict future outcomes. In the context of public audits, these techniques can be employed to identify patterns that may indicate potential risks or compliance issues. By analysing past audit findings, transaction histories, and operational data, AI systems can learn what constitutes normal behaviour for an organisation and flag deviations that may require further investigation. Historical Data Analysis, Current Trend Monitoring, and Pattern Recognition are methods for Forecasting Potential Risks and Resource Allocation, Timely interventions and enhanced audit planning are proactive problem-addressing methods.
This proactive approach not only enhances the effectiveness of audits but also strengthens organizational integrity by allowing for timely interventions that prevent problems from escalating. As public sector entities continue to embrace these technologies, they stand to benefit from improved efficiency, accuracy and overall audit quality.
9. Comprehensive Reporting
AI can significantly enhance the generation of detailed, data-driven audit reports, making it easier for stakeholders to understand findings and take necessary actions based on comprehensive insights.
AI technologies are adept at processing vast amounts of financial and operational data quickly and efficiently. Traditional auditing methods often struggle with the sheer volume of data generated by organisations, which can lead to incomplete analyses. AI can analyse entire datasets rather than relying on sampling, ensuring that no critical information is overlooked. This comprehensive analysis provides a more accurate picture of an organisation’s financial health and operational integrity.
ML algorithms excel at identifying patterns and anomalies within data. By learning from historical data, AI can detect irregularities that may indicate fraud, errors, or compliance issues. For instance, if an unusual transaction pattern emerges, AI can flag this for further investigation, ensuring that auditors focus on high-risk areas. This capability enhances the quality of audit findings and supports the generation of detailed reports that highlight significant issues.
These advancements make it easier for stakeholders to understand audit findings and take necessary actions based on clear, actionable information. As AI continues to evolve in the auditing field, its ability to transform complex data into meaningful insights will become increasingly vital for effective decision-making in public audit institutions.
Further, AI and ML will be helpful for continuous monitoring, resource optimisation, scalability, customisation, transparency and trust development.
The integration of AI within the Auditor General of Pakistan marks a significant step towards modernising public sector auditing. By leveraging advanced technologies for data analysis, risk assessment and reporting, the institution aims to enhance its effectiveness in safeguarding public resources and ensuring accountability in government operations. As these technologies evolve, continued investment in training and infrastructure will be essential for realising their full potential in improving public financial management.
Challenges of AI and Machine Learning in Public Sector Auditing
i. Security and Privacy of Data
The use of AI raises concerns about the handling of sensitive personal data. Ensuring compliance with regulations like the General Data Protection Regulation (GDPR) is critical to prevent misuse of information.
Public auditing often involves examining financial records, transactions, and other data to ensure accuracy, transparency and accountability in government or organisational operations. AI tools are increasingly being used to process large volumes of data efficiently, detect irregularities and identify patterns that may require further investigation.
TheGDPR is a set of privacy and security regulations in the European Union designed to protect individuals' personal data. Under GDPR, organizations must ensure that personal data is processed lawfully, transparently and securely. This includes having mechanisms to protect data from unauthorized access, ensuring it is used only for its intended purpose and giving individuals control over their own data.
One of the main issues with AI in auditing is that it often requires access to large datasets, which may include sensitive personal information like names, addresses, financial details, and other private data. The use of AI could raise privacy concerns because, if mishandled, this data could be exposed, misused, or accessed by unauthorised parties.
Misuse of sensitive data could include data breaches, unauthorised access or the use of personal information for purposes beyond what was initially intended. By ensuring AI systems comply with GDPR or similar data protection regulations, organisations can reduce the risk of such misuse, safeguard people's privacy, and avoid significant legal and reputational consequences.
ii. Algorithmic Bias
There is a risk that AI systems may perpetuate or even exacerbate existing biases if trained on flawed datasets. This could lead to discriminatory outcomes in public service delivery. MI &ML may lead to algorithmic bias, which is a significant concern when it comes to fairness and equity in public service delivery. Algorithmic bias refers to systematic and unfair discrimination that occurs when an AI or ML system produces outcomes that are prejudiced due to the data on which it was trained. These biases can manifest in various ways, such as favouring certain demographic groups over others or reinforcing existing inequalities.
AI and ML algorithms learn from historical data. If these datasets contain inherent biases (such as underrepresentation of certain groups or skewed data points), the algorithm may "learn" those biases and replicate them in its decision-making process. If the data used to train an AI system in public auditing reflects past prejudices or social inequalities, such as biased hiring practices or discriminatory policies, the AI may perpetuate these same biases in its analysis. An example could be an AI system that analyses financial data to detect fraud, where certain demographic groups or geographic areas were historically scrutinised more intensively. If the system is trained on this biased data, it might unfairly target those groups, even if they are not more likely to commit fraud.
Algorithmic bias doesn't just perpetuate past biases, it can exacerbate them, if AI systems are deployed to automate auditing or decision-making in public services without sufficient checks and balances, the flawed patterns in data can result in more severe and widespread impacts.
iii. Deficiency of Expertise
The lack of specialized knowledge among auditors to effectively evaluate and audit AI systems is a challenge. This gap can lead to inefficiencies, errors, and potential risks in auditing AI-driven processes. AI and ML systems are inherently complex and require a specific set of technical and analytical skills to understand, assess, and audit effectively. Unlike traditional financial systems or processes, AI systems are driven by algorithms, data models, and automated decision-making processes that are often difficult to interpret and understand without specialised knowledge.
Generally, Auditors trained in traditional financial auditing may lack the expertise to assess the underlying models, such as supervised vs. unsupervised learning, neural networks or decision trees. Understanding how these models work, how they process data, and how they make predictions is critical for auditing the outcomes they generate.
AI systems depend heavily on data. Public auditors need to understand how data is collected, processed, and fed into the system. They must be able to evaluate whether the data is biased, incomplete, or unrepresentative, which requires knowledge of data preprocessing, feature engineering, and validation techniques.
Many AI systems operate as "black boxes," meaning their decision-making process is not easily explainable. Traditional auditors may not have the technical capacity to understand why an AI model arrived at a particular decision. Without the ability to interpret AI’s decisions or the underlying processes, auditors might miss critical flaws or biases in the system.
The gap in specialised knowledge among public auditors regarding AI and ML systems is a significant barrier to effective auditing and oversight. As AI becomes more integrated into public services, auditors must be equipped with the skills and understanding necessary to evaluate these systems thoroughly and fairly. This requires robust training programs that cover the technical, ethical, and regulatory aspects of AI, ensuring that public auditors can uphold their role in ensuring transparency, fairness, and accountability in the use of AI in public sector operations. Without this training, there is a risk that AI systems will be implemented without proper scrutiny, leading to poor decision-making, ethical violations, and a loss of public trust.
iv. Governance Issues
The rapid deployment of AI and ML in public auditing presents several governance challenges. These technologies, while offering efficiency and the ability to analyse vast amounts of data, also introduce risks that need to be managed through robust governance frameworks. The primary concerns around AI and ML in auditing include accountability, transparency, fairness, and oversight.
AI and ML models often are not easily understood or interpretable by human auditors. This opacity can create challenges in public auditing, where transparency is crucial for accountability to the public, stakeholders, and oversight bodies. If AI systems make auditing decisions without clear explanations, it can undermine trust in the audit process and prevent auditors from identifying and correcting errors or biases.
In public auditing, decisions made on financial statements, compliance, or risk assessments have far-reaching implications. If these decisions are based on an AI model whose reasoning is not fully understood or disclosed, it could raise concerns about fairness, bias and the overall integrity of the audit process. Without transparency, stakeholders may be unable to verify or challenge the outcomes of an audit, which could compromise the democratic accountability of public institutions.
AI and ML can automate complex aspects of the auditing process, but this automation may blur the lines of accountability. Public audits must ensure that there are clear lines of accountability, especially when public funds and the integrity of government financial reporting are at stake. If an AI system fails to detect fraud or inadvertently introduces errors, pinpointing the responsible party becomes more complex. This may hinder proper accountability and could lead to public distrust in the auditing process.
The use of AI in public auditing may also raise ethical questions regarding privacy, surveillance, and the control of sensitive financial data. AI systems require large amounts of data to function effectively, but this data may include sensitive information that, if misused or improperly secured, could lead to violations of privacy or security breaches. Public confidence in the integrity of audits is critical. If the use of AI in auditing is perceived as overly invasive or as compromising the confidentiality of sensitive information, public trust could be undermined. This could lead to reluctance among stakeholders to accept the findings of AI-assisted audits or to push for more stringent regulations that hinder the deployment of AI tools in auditing.
V. Dependence on Technology
Over-reliance on automated systems could undermine the auditor's judgment, potentially leading to oversight failures if not managed properly. One of the key challenges that arise is the risk of over-dependence on these automated systems. This issue can undermine the auditor’s judgment and lead to oversight failures, especially if the integration of AI and ML is not properly managed within the auditing process. Let’s explore why this is a concern and provide a justification for the need to manage this dependency.
AI and ML models are designed to process large amounts of data quickly and identify patterns or anomalies that might be difficult for humans to detect. While this is a clear advantage, over-reliance on these systems could erode the role of human judgment in auditing. Auditors may begin to defer too heavily to the conclusions drawn by AI models, even in situations where human intuition, experience and professional scepticism are crucial.
Auditing is not just a mechanical process of verifying numbers, it requires a deep understanding of the context, risks, and judgment in assessing the fairness and accuracy of financial statements. Human auditors use their professional judgment to question anomalies, apply scepticism, and identify inconsistencies that AI may not flag, especially when the anomalies are subtle or non-quantitative. If auditors become overly dependent on automated systems, they may fail to exercise this necessary judgment, leading to significant oversight failures. This could result in undetected fraud, misreporting, or inefficiencies that AI models might not recognise due to their lack of contextual understanding.
Auditors rely on their ability to think critically and adapt to new and unforeseen situations, especially in cases of fraud or financial misstatement that haven't been encountered before. An over-dependence on AI may reduce an auditor's ability to recognise these novel issues. If the auditors blindly trust the AI’s output, there is a risk that new forms of fraud or errors could go unnoticed. AI systems, though powerful, are inherently limited to the scope of their training data and may lack the flexibility to identify emerging threats or anomalies that deviate from established patterns.
Public auditors are responsible for ensuring the accuracy and reliability of financial reporting, often involving millions of taxpayer dollars. If auditors become overly reliant on AI without critically evaluating its results, they may fail to detect errors or inaccuracies in the automated process. While AI can process vast amounts of data efficiently, it may still make mistakes such as misclassifying transactions or failing to flag emerging risks that a skilled auditor might catch through careful review. An over-reliance on AI can lead to a dangerous false sense of security, where human oversight is minimised, potentially resulting in undetected risks or errors in public audits.
Conclusion
Pakistan, like many countries, is actively exploring and integrating AI and ML to harness its transformative capabilities. AI and ML are reshaping industries and daily operations in both public and private sectors, driving efficiency, fostering innovation and enhancing human life. Pakistan is making significant strides in leveraging AI to improve governance and service delivery within its public sector. The country is keen on using AI to modernize administrative functions, improve public services and tackle complex challenges. However, realising AI’s full potential in governance requires overcoming barriers such as limited infrastructure, a need for skilled talent and addressing concerns about ethics and data privacy.
The integration of AI in Pakistan's public sector faces challenges, its potential to revolutionize sectors like auditing, accounting and governance is immense. By addressing these obstacles, the public sector can unlock AI's and ML's transformative power, ensuring better outcomes for its citizens and its public institutions.
Auditing ensures the accuracy and integrity of financial systems and AI's ability to sift through large volumes of data with precision makes it a valuable tool for enhancing transparency. By automating routine tasks, AI can free up auditors to focus on other emerging areas and also help to formulate more complex analyses.
AI can examine financial statements, detect fraud, improve decision-making processes and reduce costs. While the benefits are evident, challenges such as data security concerns and the potential for algorithmic biases present obstacles to full adoption. Ensuring that AI and ML systems are transparent, fair and secure is crucial, especially as the systems process sensitive financial data. Despite these hurdles, the advantages of AI and ML in the sector of audit and accounts are undeniable and its integration is crucial to enhancing transparency and efficiency.
References:
i. M. Brundage et al. (2020): Toward Trustworthy AI Development: Mechanisms for Supporting Verifiable Claims, https://arxiv.org/abs/2004.07213.
ii. Veale, M., & Borgesius, F. Z. (2022). Demystifying the Draft EU Artificial Intelligence Act. Computer Law Review International. https://doi.org/10.9785/cri-2021-22040
iii. U. von der Leyen (2019): https://g8fip1kplyr33r3krz5b97d1-wpengine.netdna-ssl.com/wp- content/uploads/2019/07/190714-Letter-Candidate-RENEW-1.pdf.
iv. High-Level Expert Group on AI (2019): Ethics Guidelines for Trustworthy Artificial Intelligence, https://ec.europa.eu/digital-single-market/en/news/ethics-guidelines- trustworthy-ai.
v. ACM Conference on Fairness, Accountability, and Transparency (ACM FAccT), https://facctconference.org/.
vi. D. Raji et al. (2020): Closing the AI Accountability Gap: Defining an End-to-End Framework for Internal Algorithmic Auditing,doi: https://doi.org/10.1145/3351095.3372873
vii. https://www.pc.gov.pk/uploads/vision2025/Pakistan-Vision-2025.pdf
viii. https://eur-lex.europa.eu/legal-content/EN/TXT/PDF/?uri=CELEX:32016R0679
ix. https://www.thenews.com.pk/print/306187-govt-allocates-rs1-1-billion-for-artificial- intelligence-projects
x. https://intosaijournal.org/journal-entry/auditing-machine-learning-algorithms/
xi. https://intosaijournal.org/journal-entry/the-future-of-public-sector-auditing-living-in-times- of-change/
xii. https://moitt.gov.pk/SiteImage/Misc/files/National%20AI%20Policy%20Consultation%20Dr aft%20V1.pdf