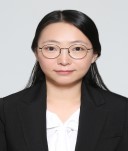
Ms. Hur Soo jeong
Senior Researcher
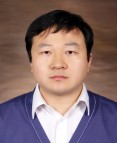
Mr. YUN Il gi
Senior Auditor
This research aims to provide foundational data for developing audit techniques in the coming years for AI-based informationsystems adoptedinthepublic sectorby analyzingrelevant issues aroundactual casesof adoption, as well as the audit methods employed in them, within South Korea (hereinafter referred to as ‘Korea’).
- The trend of AI-related policies and current status: This research analyzes the trends and details of adoption of AI technologiesby the Koreanpublic sector as well as withinrelevantnationalpolicies — i.e., the economic stimulusplans through promoting AI technologies, national strategies for AI, digital new deals, etc. Additionally, it includes an analysis of the current status of AI-based information systems either established or ordered for a contract between 2017 and 2021.
- Issues and methodologies of auditing AI-based information systems: Based on reports and academic journals producedhome andabroadon adoptingAI systems in thepublic sector, andrelevant audit frameworks1 , this research demonstrates analyses of: (a) current challenges and risk factors, and (b) sets of audit questionnaires and methodologies proposed by supreme audit institutions (SAIs) of other countries.
Considering the lifecycle of AI information systems, this research brings a light to the entire phases of adopting AI information systems — namely, from (a) establishing plans for investment and utilization (designing phase), (b) establishing the system (development phase), and (c) operating the AI-based information systems (deployment, continuous monitoring phase). It also looks into (d) ethical problems that might arise throughout the entire phases from development to utilization.
2. AI-related Policy and the Status of Investment on Informatization(1) Current status of AI policies
- The development of AI-based information system: Outside of Korea, countries take a cyclical approach to design, development, adoption, and monitoring stages of the AI information system. In contrast, however, Korea adopted a deliberation-based decision-making process, which, unlike the agile cyclical approach, is unsuitable for a quick decision-making, as it requires approval process for making any changes between different stages.
- Implementation status of AI policies: With the launch of the Presidential Committee on the Fourth Industrial Revolution, the Korean government has shown an increasing interest in developing AI infrastructure as well as establishing AI-based smart government, as envisaged in the National Strategy for AI (in February 2019) and the Digital New Deal Policy (in July 2020).
When it comes to adopting AI systems, ethical issues are one thing to consider when dealing with data and writing algorithms. Thus, the Korean government established standards, entitled Ethical Guidelines for AI and the Strategies for Reliable AI Implementation, both of which emphasize safe utilization of reliable AI systems.
Meanwhile, in order to ensure reliability of AI system and minimize its adverse impact, many countries are also establishingregulationson various aspectsof AI adoption: ethicsof AI, legalizationof right for request explanationon AI-powered decision, and safe utilization of AI system. In particular, the European Commission suggests a number of ways to manage different groups of risks associated with AI, each of which is categorized for different risk levels.
(2) Adoption status of AI-based information systems
- Adoption status: From 2017 to August 2021, a total of 117 contracts were signed for establishing AI information systems, displaying a sharp increase since 2020 (43 contracts signed in 2020 and 31 in 2021). In 2017 and 2018, the National InformationSocietyAgency took charge,onbehalfof contractorpublic agencies,ofplacingorders as well as managing implementation of such contracts. Afterwards, however, public agencies gradually began to place orders autonomously.
- Areas to adopt AI system: AI-based information systems have been adopted widely across various areas, from such lowest-risk (non-regulatory) areas as big data analysis of spam mails, to those high-risk areas that require thorough management and other compliance conditions, like detecting potential signals of criminal acts and job matching, as categorizedby theEuropean Commission. However, Korea has yet to establish regulations ondistinguishingdegrees of risk in using AI.
It refers to the audit guidelines indicated in audit frameworks developed by the SAIs of the UK, the US, the Netherlands and Norway.
- Adoption plan: For this research, various Request for Proposals (RFP)* were reviewed. The results showed that when adesignatedthirdparty for contract, i.e., the National InformationSociety Agency, was commissionedto make a contract on behalf of public agencies, performance indicators were clearly outlined in the RFP. However, when public institutions began to make contracts themselves, they either did not specify performance indicators in their RFPs, or just touched upon satisfactory conditions, not considering conditions for transparency, explainability, and ethical standards in the document.
- Relevant regulations and output: There are multiple different regulations stipulating on writing of RFP and managing AI projects. Furthermore, there is no consistent guideline for defining outputs to be achieved from AI systems. Alternatively, for each of different situations, different regulations are applied, and targeted output of information system or software development are all different, as well.
3. Audit Issues and Approaches of AI-based Information System(1) Establishment of Plans for Investment and Utilization of AI
A. Governance of information system establishment and operation - Problems and risk factors: When establishing AI-based information system, if governance structure does not engage participation of various stakeholders and public agencies placing such orders lack a certain degree of expertise on AI systems, many problems can occur: for example, transparency of the AI system and its explainability may be undermined, the cost for building the system may rise, and the system may be developed in a different direction than it was intended.
- Tips for audit: i. Engagement of various stakeholders: It is essential to check whether a range of stakeholders had been engaged in establishing the AI information system concerned. Various means can be employed; for instance, identifying stakeholders, reviewing the documents stating the activities and methodologies of the stakeholders engaged in the process of system development, and conducting interviews.
ii. Ensuring expertise: It is advisable to check whether public institutions have reserved a pool of AI experts for the process of AI adoption, and provided necessary training to them. For this, it may be worthwhile to review the professional competencies of an AI expert, established guidelines for eligibility requirements, and the documents on recruitment of AI personnel together with relevant training records. Conducting an interview with persons from human resource department would be a good option, as well.
iii. Managing external vendors:When making a contract with a private AI system provider, it is important to check the propriety of oversight performed by the public institution. Worthy to review would include contract(s), a system management plan, and a follow-up plan for managing project outputs.
B. Appropriateness of investment plan
-Problems andrisk factors: In case an AI system is adopted without sufficient review of necessity andapplicability, or specific plan for its establishment, it might cause unwanted results, making the system inefficient. The AI system may be developed in a way that does not serve the original needs of public institutions.
Furthermore, when the purpose of adopting a certain AI system is not explained clearly to the vendor, and specifications tobe appliedto the system are not well-defined, it may also leadto inefficiencies in various forms, such as low-quality of the system or inability to fulfill the originally intended purpose.
- Tips for audit:
i. Checking appropriateness of adopting AI system: It is essential to check,first, whether there has been a preliminary review of cost effectiveness when adopting an AI system through, for example, the results of cost-benefit analysis.
ii. Checking plans for establishing AI system: It is, then, useful to look into documents related to development of AI system, with a focus on the purpose of adoption, clearly defined objectives in consistency, and specifications for integrating sufficient resources, and the anticipated outcomes of adopting the AI system. In other words, it is recommended to check up the public institution’s resources for developing an AI system, i.e., whether sufficient amount of usable data has been made available.
* Translator’s note: It is a business document in which public agencies describe conditions of project to solicit bidders from external vendors.
iii. Mechanism for ensuring performance: Through analyzing documents on the performance of the pre-set system model, specification of performance indicators, as well as conducting interviews with planners and programmers, it is necessary to ensure whether performance indicators have been established for verifying the performance of the system. If established, it is worth checking the appropriateness, specificity, and consistency of the indicators.
(2) Establishment of Information Systems
A. Appropriateness of data utilisation and processing
- Problems and risk factors:When source data is inappropriate, and such data is processed, it may harm the reliability of an AI system's performance, as such data would be of low quality. Besides, when data or variables are to be manipulated for the sake of improving AI performance, there is a risk of undermining important values such as the distinctiveness of the data/variables, explainability, and protection of personal information.
In particular, ambiguous distinctions between training dataset and test dataset, as well as inadequate data preprocessing and data imputation can lead to problems, such as decline of applicability of an AI system model due to low reproducibility, bias in outcomes, and even contradictory results. Furthermore, when developing a model, selecting sensitive variables can prompt ethical problems, as well.
- Tips for audit:
i. Ensuring quality data: It is necessary to check whether there have been efforts to ensure data quality by reviewing documents related to data used for developing the AI system (variables and sources) and reviewing technical reports on methodologies of processing data imputation.
ii. Checking contradictory values: Through checking a review report on the possible contradiction between system performance and values to be pursued, which is usually devised during the development phase of an AI system, it is also significant to ensure that there have been efforts to: (a) leverage technology for detecting or minimising contradictory values, (b) include a balance point in the system by embedding conditions for it, and (c) set up conditions for monitoring.
B. Verification of system performance and establishment of test framework
- Problems and risk factors: If performance verification is not conducted properly, it may result in inefficiencies in operating the AI system because the system performance may be degraded and thus, additional contracts may be needed for maintenance.
To be more specific, using inefficient algorithms during system development by writing it too complicated to be shared by general users can lead to additional costs for performance improvement. Additionally, if performance verification is not conducted to a sufficient degree, the following problems can occur: the AI system may not be able to serve its intended purpose; the AI system may not be as reliable as expected due to weakened performance; thereby, decrease in usage of it.
To prevent these problems, it is important to disclose the results of system establishment and secure an objective review by a third party. However, if the system is developed by an external provider, disclosing this information may be challenging.
- Tips for audit:
i. Reviewing developed algorithm: It is necessary to review whether: unnecessary data have been applied, algorithmic transparency has been secured, the system model was designed too complicated, or the code used has been developed only to be understood or executed by a specific group(s).
If it is difficult for an auditor to review directly the algorithm structure and the original data for development code, (s)he is recommended to do it through available internal data.
ii. Reviewing methods of verifying the performance of the AI system model: It is important to conduct a comparative analysis of documented records on the process of AI system development and established performance objectives. This will help ensure that the AI system model has been developed to serve the purpose of the system as well as its performance indicators. It is alsoadvisable toexamine whether appropriate statisticalmethods havebeen appliedto verify the applicability of the system model.
(3) Operation of Information System
A. Management of system operation and feedback
- Problems and risk factors: Capacity of an AI system can get degraded when operation of an AI system is not managed properly. In other words, there should be periodic feedbacks based on the results of system operation, sporadic check-ups in response to unexpected environmental changes, and retraining of the AI system.
As AI systems are toevolveover timeby itsnature, it takes consistenthumanefforts formaintainingsystemcapacity. It should include both periodic feedbacks and infrequent feedbacks in response to environmental changes, i.e., system-related policies.
- Tips for audit: It would be practical to: (a) check various documents on plans for periodic feedback, frequency of monitoring and its rational, explanation for detecting changes in AI system model, records of expert’s analysis of performance verification; and (b) conduct interviews with relevant staff.
B. Cybersecurity
-Problems andrisk factors:AI informationsystems standtoprocess a large amountofdata automatically inreal time. Thus, the more the amount of data is utilized, the wider area become target for cyberattacks. It is often so swift that data in the AI system gets easily distorted, and the distorted data brings distorted decision-making.
In particular, distortion of the training dataset used for an AI system can alter the algorithm structure, obviously leading to distortion in results, which would then cause serious overall impact on reliability of the AI system.
- Tips for audit: Effective methods to prevent AI-targeted cyberattacks have yet to be specified. However, it is advisable to check whether response and prevention plans for cybersecurity threats are in place and being implemented.
- On the stage of planning investment and utilization:
- On the stages of AI information System development and operation:
- On ethical aspects:
As revealedin real audit cases in Korea, ambiguousgoals andperformance indicatorsofAI adoption act asobstacles in assessing the success of system establishment. It is, therefore, necessary to ensure that the purpose and objectives of the system establishment be clearly defined.
Also, it is important to verify whether a detailed plan for data utilization and algorithm development—one of key distinctions of AI systems from other information systems—exists from the planning stage.
In addition, there is a need to check whether a governance structure involving diverse experts is in place to gather various input from them, and whether there is a clear plan for managing and overseeing contracts with external vendors through checking AI-related laws, regulations, and guidelines.
It is necessary to review documents, such as up-to-date enterprise architecture materials or reports on algorithm implementation detailing data processing steps to ensure data quality, check representativeness and/or bias, as well as data security and privacy concerns in AI information systems. This review should include assessing data sources, checking data processing and imputation.
In order to verify that an AI system has achieved intended outcomes, it is important to check whether there are plans for constant and regular monitoring of AI system, and whether a mechanism for constant feedback loop based on the results of AI system operation had been established.
According to the current status of contracts for establishing AI information systems, the focus has been primarily on the utilization and effectiveness of these systems, rather than on ethical criteria, such as transparency and explainability.
However, as far as adopting AI information systems into the public sector is concerned, consideration of ethical standards shouldtakeprecedence above all else.Tothis end, it is necessary tocheck whether efforts arebeingmade to enhance transparency and explainability of AI system by providing various stakeholders, including users and regulatory agencies, with access to the information regarding the design, operation, and limitations of the AI information system.
In future audits, it will be necessary to check whether the AI information systems being implemented in Korea are achieving their intended outcomes, through looking into the results of adopted AI system performance. For doing so, it would be beneficial to take into consideration the audit implications in this research and the audit frameworks for AI information systems from SAIs of other countries, such as the Government Accountability Office of the United States.