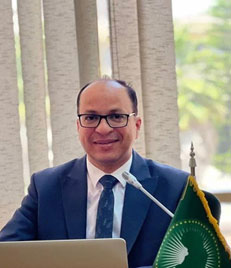
Dr. Samy Ali Mohamed,
General Manager at SAI - Egypt
Abstract:
Artificial Intelligence (AI) and Machine Learning (ML) have created a revolution in public sector auditing, offering unprecedented opportunities for enhancing accountability, efficiency, and fraud detection. These technologies enable auditors to analyse big data, improve risk assessments, and detect variations in financial and operational processes. However, using AI and ML in audits is not free of challenges. There are many concerns and complications that may be involved in this matter about data quality, explainability, resource demands, and the skills gap.
Strategic recommendations for successfully adopting AI technologies include establishing clear governance frameworks, investing in workforce development, implementing robust data management practices, and addressing ethical considerations. Public sector institutions must adopt cautious, responsible approach, emphasising transparency and public trust to harness AI's full potential while mitigating risks.
Introduction:
In an era of technological innovation, AI and ML are reshaping how governments deliver services, manage operations, and ensure transparency. Supreme Audit Institutions (SAIs) have a unique status enabling them to leverage these technologies to enhance accountability, efficiency, and insight within the public sector. However, integrating AI and ML into auditing also presents various challenges that must be carefully navigated.
AI and ML are transformative fields poised to shape the future of the auditing profession. ML focuses on enabling systems to perform tasks that emulate human auditor intelligence. The evolution of auditing will extend beyond simple automation using Robotic Process Automation (RPA), as, machines will progressively learn from auditing processes and apply human-like intelligence to analyse similar datasets.
1. Understanding What is AI?
Artificial Intelligence refers to the broader concept of machines or systems that can simulate human intelligence to perform tasks autonomously. These systems can reason, learn, perceive, and make decisions like humans. AI encompasses a vast range of subset techniques, including Machine Learning (ML), Neural Networks (NN), and Natural Language Processing (NLP).
Below are some of the major subsets of artificial intelligence:
Machine Learning (ML): focuses on developing algorithms and statistical models that enable computer systems to perform tasks without explicit programming. The primary goal of machine learning is to allow machines to learn patterns and make decisions based on data.
Neural Network(s) (NN): focuses on AI models inspired by the human brain's structure. These networks consist of layers of interconnected nodes, each layer contributing to the model’s ability to understand increasingly complex features in the data, which is called deep learning which is a class of multi-layer neural networks. Deep learning has succeeded in tasks like image recognition and natural language processing.
Natural Language Processing (NLP): focuses on enabling machines to understand, interpret, and generate human language. This subset is crucial for applications like chatbots, language translation, sentiment analysis, and voice recognition.
2. Opportunities of AI and ML in Public Sector Audit
- Enhance Data Analytics: AI tools for data analysis are software, programs, or libraries designed to streamline and empower different stages of the data analytical process. Such tools can automate various analytical tasks, such as data collection from multiple sources, organising the data, predictive modelling, segmentation, etc., using machine and deep learning algorithms. In addition, Machine learning algorithms can identify patterns, anomalies, and trends in financial statements, helping auditors focus on areas that require closer examination. AI-powered analysis can be a game-changer for auditors, enabling them to rapidly process and extract insights from vast troves of financial and operational data. By leveraging the speed and precision of AI, auditors can discover patterns, trends, and anomalies that were previously unnoticed. For example: The UK’s National Audit Office (NAO) used data analytics tools to examine over £100 billion in transactions, uncovering inefficiencies and improving financial oversight.
- Improve Risk Assessment Process: AI-powered risk assessment is the use of artificial intelligence to identify, evaluate, and prioritize potential risks within the organization. AI algorithms can sort mountains of financial records, operational data, and other information sources to uncover patterns, trends, and anomalies that may signal potential risks. By prioritising risks based on their likelihood and potential impact, organisations can allocate resources more effectively and implement targeted mitigation strategies to safeguard their operations and financial status. Also, by leveraging predictive analytics, SAIs can prioritise high-risk areas for audits. ML models trained on historical audit data can identify departments or projects most likely to exhibit noncompliance or inefficiencies.
- Strengthened Fraud Detection: It is the process of identifying dishonest or illegal activities, like stealing money or manipulating information, usually in financial transactions or business operations. By leveraging machine learning algorithms, AI-based systems can rapidly analyse vast amounts of financial data, transaction records, and other relevant information to identify anomalies, patterns, and red flags that may indicate the presence of fraudulent activities. AI-powered fraud detection can uncover subtle discrepancies and suspicious transactions that may have previously slipped through the cracks, allowing organisations to take proactive measures to address these issues and mitigate the associated risks.
- Continuous Compliance Monitoring: It is the process of regularly checking and assessing whether an organisation is adhering to laws, regulations, standards, and internal policies. It involves evaluating the company's practices and procedures to ensure they meet legal and regulatory requirements, industry standards, and organisational policies. AI systems can continuously monitor transactions, activities, and operations in real-time, ensuring they align with legal and regulatory requirements as well as internal policies. This allows for immediate detection of non-compliance issues.
- Enabling NLP is a field of artificial intelligence that focuses on how computers can understand, interpret, and respond to human language. It's what enables machines to read, listen, and even generate text in a way that feels natural to us. NLP allows AI tools to analyse unstructured text data. In data analysis, NLP can add value to the auditors by extracting insights from different audit reports across years, sectors, or organisations. By analysing large volumes of unstructured text data, NLP can help identify patterns, trends, and anomalies that may not be easily detected through manual review. NLP could be used to analyse the wording and structure of audit reports to detect potential areas of risk. It could also be leveraged to consolidate and summarise key findings and recommendations from multiple reports, providing auditors with a high-level overview of the organisation's performance and compliance.
3. Challenges of implementing AI and ML in Public Sector audit
While AI offers many advantages, there are several limitations and challenges associated with its use. These limitations span technical, ethical, and social aspects. Below are some key challenges faced when using AI systems:
- Data Requirements: AI systems, particularly machine learning models, rely heavily on large amounts of data for training. If the data is incomplete, biased, or of poor quality, the AI's predictions and outputs will be defective. For example, facial recognition technologies have shown higher error rates for certain ethnicities due to biased data.
- Accountability and Transparency (Black Box Problem): Many AI models, especially deep learning algorithms, function as "black boxes," meaning their decision-making processes are not easily understood or explained. This lack of transparency makes it difficult to trust AI decisions, particularly in sensitive applications like healthcare or finance. Also,when AI makes decisions that affect people’s lives (e.g., loan approvals, hiring), there’s a challenge or lack of accountability in identifying responsibility if something happens, especially in complex models where human oversight is limited.
- Security Concerns Due to Cyber Attacks: AI systems, especially those used in critical infrastructures like healthcare and finance, can be targeted by cyberattacks. Hackers can manipulate AI algorithms or inject false data to alter their output, compromising the system’s integrity.
- High Costs and Resource Intensity: Development and Maintenance Costs of Implementing AI systems can be expensive, requiring significant investments in technology infrastructure, data storage, and skilled personnel. Smaller organisations may struggle to afford these resources. Also, Energy Consumption of AI models, especially deep learning models, require enormous computational power, which leads to high energy consumption. This raises concerns about the environmental impact of large-scale AI systems.
- Technical Expertise: AI implementation requires expertise in data science, programming, machine learning models, and advanced analytics. Auditors often lack these specialised skills, as their training typically focuses on financial, performance, or compliance auditing rather than technological or data-driven methods.
- Developing a Clear Vision and Objectives: Set specific goals for the application of AI and ML that are bounded by the overall organizational aims.
- Investing in Training and Workforce Development: It should also be mandatory to offer training to teach staff members about the technicalities and the unethical use of AI tools.
- Implementing Robust Data Management Practices: Promote compliance to data accuracy and security through conformity to industry standards of data management.
- Establishing Ethical Guidelines and Governance Frameworks: Establish rules regarding bias and transparency of one’s position when overseeing and managing AI projects.
- Addressing Data Privacy and Security Concerns: Configure data security and create guidelines on acceptable use of information to protect the data.
References:
(1) University of Illinois Chicago, May 2024, what is (AI) Artificial Intelligence? https://meng.uic.edu/news-stories/ai-artificial-intelligence-what-is-the-definition-of-ai-and-how-does-ai-work/
(2) Dr.Harib Saeed Amimi “ The future of public sector Auditing: Living in the times of change”https://intosaijournal.org/journal-entry/the-future-of-public-sector-auditing-living-in-times-of-change/
(3) World Bank 2020. Artificial Intelligence in the Public Sector | Maximizing Opportunities, Managing Risks. EFI Insight-Governance. Washington, DC: World Bank.
(4) AI in government: AI law, use cases, and challenges, By Pluralsight Content Team, April 2024, https://www.pluralsight.com/resources/blog/ai-and-data/ai-government-public-sector#ai-challenges- government