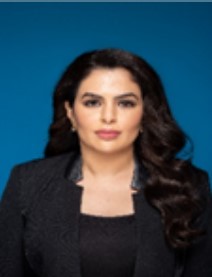
Ms. May Abdulaziz Al-Roomi
May Abdulaziz Al-Roomi is a Senior Information Systems Auditor at the State Audit Bureau of Kuwait
This article describes how AI and ML are reshaping the auditing process of the public sector. While these new concepts have brought in unprecedented opportunities to improve audit efficiency, enhance fraud detection, and streamline compliance procedures, they also introduce significant challenges that need to be fully addresses including data privacy concerns, ethical implications, and associated skills development. This article elaborates on how AI and ML affect auditing practices in government sectors and public institutions by considering present applications and future trends.
Introduction1.1 Background
Auditing has become more critical than ever in the public sector. The finances of government and public institutions must be managed prudently, services delivered efficiently, and compliance with regulations strictly enforced. Traditional auditing methods, though quite effective in several aspects, are labor-intensive and are sometimes limited in scope. Moreover, they are more prone to human mistakes. AI refers to the replication of human intelligence in machines so that these machines can effectively perform tasks that fundamentally require human cognition, such as learning, problem-solving, and decision-making. Machine learning is part of AI that deals with developing systems that can learn from data, outline patterns, and thereby improve over time, all without explicit programming. These technologies can automate routine tasks in auditing, analyze largedata sets for fraud, andare far more effective than amanualprocess. Inaddition, tasks that typically take a longperiodof time canbeperformed withease insecondsby utilizing AI and ML.
1.2 Importance of Auditing in the Public Sector
Auditing is the sentinel of public sector operations, bringing transparency, accountability, and efficiency in resource allocation. The various aspects that fall under the umbrella of public sector audits are auditing of financial records, efficiency in operations, compliance to laws and regulations, and audit of performance metrics. Accuracy and integrity of these audits are of paramount importance to retain public confidence. However, traditional auditing methods often cannot disclose fraud schemes that are complex in nature, analyze huge volumes of data, or deliver real-time insights. AI and ML can contribute significantly to solve these problems, enabling auditors to devote more time to strategic decision-making and higher-risk areas, leaving repetitive tasks or the initial analysis of data to machines.
2. The Role of AI and ML in Modern Auditing2.1 Automation of Routine Tasks
Key advantages of AI in auditing mainly involve the automation of tasks that are mundane and time-consuming. An example of such applications includes AI auditing tools that carry out activities such as data entry, transaction matching, and verification involving large sets of records. This approach does not only save time but reduces possibilities of human error, and thereby, enhances the accuracy of the whole audit process. Automation can be particularly helpful in the public sector. Automating the processing of transactions allows auditors to focus on higher-level analysis and risk management, ensuring that public funds are used effectively.
2.2 Enhanced Data Analytics
AI and ML can process large volumes of data at incredible speeds, well beyond human capabilities. This is a game-changer in auditing because such algorithms are highly capable of reviewing millions ofline items within large datasets to find patterns and flag items for fraud, inefficiency, or regulatory violation. Such systems will be able to identify relationships and trends in the data that would not be immediately evident to a human auditor. For instance, ML can scan thousands of transactions in procurement processes to identify unusual patterns of bidding that would almost certainly signal collusion or corruption in the public sector. Similarly, analytics enabled by AI can provide real-time insights that is enabledby continuous real-time auditingrather than review only after theoccurrenceof the event.
2.3 Predictive Auditing
AI-powered predictive models can anticipate, with a large degree of accuracy, areas of high risk before they become problematic. Through the application of historical audit data, analysis of financial records, and other related factors, AI systems can forecast potential issues before they occur, and thereby, target auditors accordingly to channel their efforts into such areas. Predictive auditing in the public sector can be applied to determine prospective compliance risks, budget overruns, or operational inefficiencies. For example, AI can analyze public project expenditure patterns to estimate which projects are likely to overrun their budgets. This allows for proactive intervention rather than a reactive problem-solving approach. By addressing potential risks early, governments can mitigate negative outcomes, improve accountability, and enhance overall efficiency in public resource management.
3. Opportunities for the Public Sector3.1 Improved Efficiency
Public sector organizations are often constrained in resources such as personnel and time. The AI-driven audit systems accelerate audit processes through the automation of data collection and preliminary analysis. Operations that typically take several weeks can be done in a few hours, or minutes. Hence, more extensive audits can be executed within a short period of time, which helps increase the scope for more complex operations. Thus, AI-based systems will enhance the quality and effectiveness of the audit results. Furthermore, real-time monitoring with the power of AI allows for ongoing assessments rather than a review that is annual or semi-annual. The ability for continuous auditing enables identifying and resolving issues promptly, hence preventing big discrepancies over time.
3.2 Real-Time Monitoring and Continuous Auditing
One of the major advantages of utilizing AI in auditing is the real-time tracking of events related to finances and operations. AI systems allow for constant analysis of streams of data, flagging potential issues at their emergence time. This is highly significant in the public sector, which involves a large number of financial resources and sensitive information. Continuous auditing powered by AI enhances transparency because it reduces the gap between data collection and review. Public sector organizations can respond more quickly to irregularities, preventing small issues from escalating into major problems.
3.3 Enhanced Fraud Detection
One very critical area where AI and ML can make an overwhelming difference is in fraud detection. Public sector organizations, given the large amounts of finances handled and the complexity involved in the operations, are naturally amongst the biggest targets of fraudulent activities. AI systems have the capability to analyze transaction data, payroll records, procurement contracts, and other financial data for patterns that may suggest fraud. Various ML algorithms can be trained to identify fraud indicators, such as unusual spending, duplicated payments, or procurement process discrepancies. As the system processes more data over time, it becomes increasingly adept at detecting even the most complex and sophisticated fraud schemes. This proactive approach helps public sector entities safeguard public funds.
3.4 Improved Decision-Making
AI helps auditors drive active insights by providing them with all the data they need to make strategic or more-informed decisions. Auditors can focus on interpreting the data and determining the appropriate course of action, rather than spending extensive time collecting and analyzing the information themselves. Such an approach is immensely important in the public sector because decisions on areas such as resource allocation or policy development can have significant consequences for society. AI helps identify trends, predict outcomes, assess effectiveness of programs, allowing decision-makers to act proactively and with greater precision.
3.5 Cost Reduction
The implementation of AI in public sector auditing can result in substantial cost savings. By automating routine audit tasks and streamlining data analysis, AI systems reduce the need for extensive manual labor. This allows human auditors to focus on higher-value, more complex areas that require critical thinking, while the AI handles time-consuming data processing tasks. Moreover, by improving fraud detection and minimizing financial discrepancies early, AI helps public entities prevent financial losses, misallocations, and inefficiencies that would otherwise require costly interventions to resolve. Additionally, the savings accrued from AI-optimized operations can be reinvested in improving public services, providing a net benefit to society.
3.6 Scalability of Audit Operations
As the size of the public institution increases and its activities become more complex, traditional auditing methods cannot scale. AI provides a window through which large volumes of data from real-time interactions can be audited regardless of organizational size or scope. Whether it was auditing a single department or one whole governmental organization, AI systems can process millions of transactions or documents without being overwhelmed. This scalability ensures that auditing is scaled out easily to meet the expanding operations of the public sector without loss of accuracy and efficiency. This is particularly valuable for large-scale projects, whether in infrastructure development or healthcare systems, where delays or errors can have wide-ranging and significant impacts.
3.7 Data-Driven Policy Formulation
Artificial intelligence not only facilitates the audit process but also generates meaningful insights that inform policy decisions, leveragingthedata itprocesses formoredata-drivengovernance.Public sectororganizationspossess an extensive volume of data that is associated from feedback from citizens, performance of social services, financial records, and national statistics. AI is crucial in sorting all this raw information into actionable insights that help make policy decisions. For instance, predictive analytics can be used to forecast the future needs of a community, and hence guide resource allocation in health, education, or even urban development. Data-driven policies ensure that the work of the governments is relevant to the real-time needs and trends of the day, hence assuring better outcomes for the public. Thus, policies are evidence-based, not assumed.
4. Challenges in Implementing AI and ML in Public Sector Auditing4.1 Data Privacy and Security
One of the major challenges in implementing AI in the public sector is maintaining an adequate level of integrity, confidentiality and availability of data. Public organizations handle vast amounts data that involve personal information,financial transactions, and even secret correspondence.With the requirement for AI systems to access and process such data, leaks, unauthorized access, data breaches, or even misuse of such sensitive information becomes a major concern. Application of highly strict security measures in place, such as encryption, access controls, data masking and anonymization, backups and regular audits of the AI systems themselves, will only mitigate these risks to a certain extent. Privacy-related regulations, such as the General Data Protection Regulation (GDPR), must be implemented to ensure that AI systems, when processing sensitive data, maintain individuals' rights to privacy rather than compromise them.
4.2 Ethical Considerations
AI systems are only as unbiased as the data they are trained on. The risk, in public sector auditing, is that these systems could be biased towards certain results if the data they were trained on is biased historical data. For example, if past audit data has been biased towards flagging certain departments or individuals for scrutiny based on demographic factors, then the AI system will learn to replicate that same bias. Consequently, training on diverse data and regular audits for fairness and bias is a must for all AI systems. Public sector organizations must be fully transparent when arriving at certain decisions made by AI by incorporating human auditors for the final judgment.
4.3 Skill Gaps and Workforce Adaptation
Utilization of AI in auditing the public sector requires that auditors at least understand how AI and ML systems work. This would definitely demand considerable technical knowledge, and hence, most auditors will need formidable trainingonsuchskills.Public sectororganizations willhave toinvestheavily intheupskillingof their audit teams such that they become capable of using AI-driven systems. This would include training on data analytics, understanding of AI algorithms, and accurate interpretation of results presented by the AI tools. Therefore, collaboration between data scientists and auditors is essential for bridging critical gaps in technical and domain expertise.
4.4 Organizational Resistance
Another major impediment to the use of AI in the public sector is organizational resistance to change. Most public institutions are slow to adopt new approaches because of the bureaucratic nature of operations. Employee resistance is also highly possible because the audits that is typically performed by them will be performed by AI systems. To overcome such resistance, the complementary nature of AI needs to be emphasized by public sector organizations. Instead of replacing auditors, AI extends their competencies as mundane tasks will be adopted by such systems so that auditors can focus more on the complex and strategic tasks of auditing. Implementing this transformation will require clear communication and change management strategies.
4.5 Regulatory and Legal Challenges
Most AI applications within the public sector are faced with major regulatory and legal barriers. In most countries, there is a lack of clear guidelines or regulations associated with audits in the public sector that involve AI. This can create many issues and ambiguities in terms of compliance and accountability. In this regard, legal systems may lack adequate provision for addressing AI-related issues such as decision-making by AI systems, ownership of data, and legal liability. Therefore, public organizations must work with lawmakers to come up with robust legal frameworks that stipulate the use of AI, ensuring its compliance with national and international regulations.
4.6 Data Quality and Availability
AI systems rely heavily on large, high-quality datasets for accurate predictions and insights. However, in the public sector, clean and comprehensive datasets are hard to obtain. Public sector data can be incomplete, inconsistent, or even outdated, thus restricting the AI systems' performance level. Furthermore, each department may store data on different systems, making the integration of such data into an integrated AI model difficult. Therefore, assuring data quality, standardizing data formats, and improving data-sharing arrangements between public entities are essential to enhance the effective use of AI in auditing.
5. Case Studies: AI and ML in Public Sector Auditing5.1 AI in Fraud Detection in the United States
The Government Accountability Office (GAO) in the United States has used AI to improve the detection of fraud across government-funded programs, specifically in the areas of healthcare and social security. AI was used to analyze vast transactional data across different departments to identify fraud claims and patterns which have led to significant cost savings for thegovernment. AIdetectedapattern in healthcare claims from repeatproviders, raising a red flag. As a result, it uncovered a large fraud scheme involving fictitious medical service, something that would have been difficult to spot using traditional auditing methods.
5.2 Predictive Auditing in the European Union
Some EU members have already implemented predictive models enabling the auditing resources to focus their efforts on areas of high risk. In Denmark and the Netherlands, ML algorithms are used to analyze data that pertains to public spending to predict which projects are at the highest risk of budget overruns. In such case, auditors can target their efforts and resources toward these high-risk areas, preventing cost overruns before they even occur. Another example is the European Court of Auditors, using AI-powered tools to predict which funding programs are most likely to be non-compliant with relevant EU regulations. The EU thus focuses its auditing work on such priorities, achieving better compliance with fewer instances of infringement.
5.3 AI in Tax Compliance for Brazil’s Receita Federal Case
Receita Federal has introduced the use of AI and machine learning techniques in the detection of fraud. Using such algorithms for tax return data helped identify suspicious patterns, inconsistencies, and anomalies. AI was able to detect a large network of shell companies employed to avoid taxes by issuing fraudulent invoices for services never delivered. This automated auditing system brought the irregularities to light much quicker and assisted in the recovery of considerable unpaid amounts of taxes, offering a huge boost to revenue within the Brazilian government.
5.4 Machine Learning in Payroll Audits for Canada’s Phoenix System
Canada's payroll system, Phoenix, was troubled with glitches that resulted in overpayments, underpayments, and failure of required payments. The government reacted by using ML algorithms to audit the payroll system and predict future payroll issues. An AI model could cross-reference data from various departments to rapidly pinpoint the root cause of payroll errors, whether it is misclassification of employees or system misconfigurations. This predictive audit approach allowed Canada to proactively correct payroll issues and mitigate financial errors before they became systemic problems, improving payroll accuracy.
5.5 AI in Risk-Based Auditing for Australia’s Centrelink Case
Australia's Department of Human Services has incorporated AI and machine learning in Welfare payment auditing and monitoring. The Centrelink program analyzes large volumes of income reports, past payments, and other individual financial records to predict which welfare claims may be fraudulent or mistaken. An AI system highlighted a pattern of fraudulent claims for unemployment benefits which reflect false income reporting. Those suspicious cases were automatically flagged by the system for human auditors to review; a broader welfare fraud scheme was identified. This AI-powered auditing method significantly improved audit efficiency by reducing manual workload and increasing fraud detection accuracy.
5.6 AI in Contract Compliance Audits for Singapore’s Smart Nation Case
AI-powered systems are installed in Singapore for auditing contracts and vendor agreements related to a range of public sector projects. The AI system reviews the terms of contracts against actual performance data to find areas where non-compliance could arise-whether it is vendors failing to deliver on time or charging more than agreed. In one instance, the AI detected a pattern of a contractor who continuously provided low service levels but over invoiced the government. By flagging this anomaly, the system allowed auditors to act and impose further compliances, which improved contractor performance and cost savings.6. Future Outlook
6. Future Outlook6.1 Technological Advancements
The future of auditing is promising with the growing use of AI and machine learning, as these technologies continue to advance and expand their capabilities. For instance, adoption of new forms of technology such as Natural LanguageProcessing(NLP) will allow auditors torapidly review vast volumesof unstructured, text-baseddata items such as emails, contracts, and minutes of meetings to identify areas of high risk. With each evolution, AI systems will be capable of executing highly complex auditing tasks instantly, such as the performance assessment of public programs, or evaluation of the social impact of government policies. This approach allows auditors to gain a deeper and more insightful understanding of how the public sector operates.
6.2 Policy and Regulations
The integration of AI in auditing within the public sector is bound to increase continuously, and as such, governments across the globe will need to establish clear policies and regulations to govern its use, ensuring transparency, accountability, and ethical implementation. Presuming this takes place, then governments would have to engage with a myriad of ethical issues raised by AI, including but not limited to biases, job displacement, a lack of clarity within the decision-making process, data privacy, accountability, and the general undermining of human judgment and oversight. Moreover, the regulatory authorities must establish clear criteria on the use of AI in auditing, whereby audits carried out through AI techniques would be considered to meet all legal and ethical requirements. This is imperative to maintain the confidence of the public in the auditing process itself
6.3 Skills and Workforce Evolution
The increasing uses of AI and automation in auditing will not only impact how audits are performed but will also significantly affect skills and jobs within the workforce. Auditors will have to shift their focus toward higher order analysis and strategic activities as AI systems take over repetitive and data-intensive tasks. This evolution requires auditors to develop new competencies in areas like data science, machine learning, and AI oversight to effectively work with such advanced technologies.
Human auditing will increasingly be about interpreting AI's insights into meaningful actions, informed judgments, and wise strategies. Auditors will have to be proficient in understanding exactly how the AI models work, what their biases mightbe, andthe ethics in usingsuch approaches.With this, soft skills such as critical thinking, ethicaldecision making, and communication will be even more in demand when auditors must navigate their way through complex AI-driven audits and explain the implications of AIfindings to stakeholders. The organizations will, therefore, need to invest in upskilling programs to prepare their workforce with the required technical and analytical skills in this new environment. Such collaboration between AI and human auditors will be essential in ensuring that the auditing process remains robust, transparent, and aligned with legal and ethical standards.
7. ConclusionAI-powered and ML-powered will definitely transform the way public sector auditing is performed, characterized by improvements in efficiency, better fraud detection, and more rational judgments that are based on evidence. These technologies, when combined, will make audits faster and much more accurate, enabling public sector organizations to identify risks and irregularities as they occur. AI will also provide auditors with powerful tools to uncover patterns and insights that traditional methods may lack, leading to greater efficiency and reduced operational costs.
However, despite the immense potential of AI and ML, it is crucial to address the challenges to ensure proper usage. One major challenge is data privacy, particularly because many audits in the public sector involve sensitive information that must be protected from disclosure or misuse. Therefore, strong data security protocols and adherence to legal standards are essential. This also raises ethical concerns, especially with the use of AI, which, when not monitored, may lead to biased decisions. Hence, there is a need for balance, with emphasis on the transparency of AI algorithms and decision-making processes.
Theother challenge is adaptingthe workforce tothis technological shift.Withthe emergenceofAI and ML, auditors in the public sector will have to improve their skills to work effectively with these tools. Because of this, programs for training and reskilling should be instituted, so auditors may interpret insights coming out of AI, make informed judgments, and retain human judgment to maintain the integrity of the audit process. Of equal importance is considering upskilling of an organization's existing workforce to ensure seamless transitions and to maximize all benefits from AI technologies. This means that the public sector organization must actively take advantage of the opportunities afforded by AI but at the same time be aware of the potential risks. In integrating AI and ML in auditing, success will involve striking the right balance between using technology to drive efficiency on one hand and protection of ethical standards and openness of procedures so integral to the public sector on the other. As AI continues to improve, auditors will play an increasingly important role in the responsible application of such technologies in a manner that advances accountability, transparency, and integrity within public institutions.
In conclusion, while AI and ML hold significant potential to transform public sector auditing, careful consideration is essential to navigate both the benefits and challenges that come with this shift. Public sector organizations could unleash the full potential of AI in audit quality and efficiency while concurrently earning public trust by addressing issues of data privacy, ethics, and adaptation to changes in the workforce. As these technologies continue their development, auditors will also be at the forefront to ensure that AI is not just used to make processes more efficient but also uphold the principles of good governance and accountability in public institutions.
References:
- 1.GovernmentAccountability Office (2021)Artificial Intelligence:AnAccountabilityFramework forFederalAgencies and Other Entities. Available at: https://www.gao.gov/products/gao-21-519sp (Accessed: October 2024).
- 2. Brookings Institution (2021) Using AI and Machine Learning to Reduce Government Fraud. Available at: https://www.brookings.edu/research/using-ai-and-machine-learning-to-reduce-government-fraud/ (Accessed: October 2024).
- 3. Brookings Institution (2021)AI Use in GovernmentFraud Detection forAustralia’s CentrelinkProgram.Available at: https://www.brookings.edu/research/using-ai-and-machine-learning-to-reduce-government-fraud/ (Accessed: October 2024).
- 4. Microsoft (2021) How Microsoft and AI Work to Reduce Government Benefit Fraud and Error. Available at: https://www.microsoft.com/en-us/industry/blog/government/ (Accessed: October 2024).
- 5. Microsoft (2021) Transforming Contract Compliance Audits with AI in Singapore’s Smart Nation Program. Available at: https://www.microsoft.com/en-us/industry/blog/government/ (Accessed: October 2024).
- 6. Government Accountability Office (2021) Artificial Intelligence in Health Care: Benefits and Challenges of Technologies to Augment Patient Care. Available at: https://www.gao.gov/products/gao-21-7sp (Accessed: October 2024).
- 7. European Court of Auditors (2021) AI in Predictive Auditing for EU Budget and Compliance. Available at: https://www.eca.europa.eu (Accessed: October 2024).
- 8. Receita Federal (2021) Machine Learning in Tax Compliance for Brazil. Available at: https://www.gov.br/receitafederal/ (Accessed: October 2024).
- 9. Government of Canada (2021) ML and AI Applications in Payroll Audits for the Phoenix System. Available at: https://www.canada.ca/en/treasury-board-secretariat/topics/pay.html (Accessed: October 2024).
- 10. Australian Government Department of Human Services (2021) AI in Welfare Auditing: Centrelink Case Study. Available at: https://www.servicesaustralia.gov.au/centrelink (Accessed: October 2024).
- 11. Smart Nation Singapore (2021) Contract Compliance Audits Using AI in the Smart Nation Program. Available at: https://www.smartnation.gov.sg/ (Accessed: October 2024).
- 12. OECD (2020) Auditing in the Digital Age: Leveraging AI for Public Sector Audits. Available at: https://www.oecd.org (Accessed: October 2024).
- 13. IMF (2020) Harnessing AI for Effective Public Sector Audits and Fraud Detection. Available at: https://www.imf.org (Accessed: October 2024).
- 14. World Bank (2021) Artificial Intelligence for Public Accountability: Lessons for Auditors. Available at: https://www.worldbank.org (Accessed: October 2024).
- 15.European Commission (2021)AI inAuditing: Compliance withEU Regulations inPublicSectorPrograms.Available at: https://ec.europa.eu (Accessed: October 2024).
- 16. ACCA (2020) Artificial Intelligence and Machine Learning in Auditing: Opportunities for the Public Sector. Available at: https://www.accaglobal.com (Accessed: October 2024).
- 17. Deloitte Insights (2021) AI and Machine Learning: Game Changers for Public Sector Audits. Available at: https://www2.deloitte.com/global/en/insights.html (Accessed: October 2024).
- 18. PwC Global (2021) The Impact of AI on Public Sector Auditing. Available at: https://www.pwc.com/gx/en/services/audit.html (Accessed: October 2024).
- 19. KPMG International (2021) Ethics and AI: The Challenges of Public Sector Implementation. Available at: https://home.kpmg/xx/en/home/insights.html (Accessed: October 2024).
- 20. McKinsey & Company (2021) Artificial Intelligence in Government Auditing: Reducing Fraud and Optimizing Public Resources. Available at: https://www.mckinsey.com/business-functions/risk-and-resilience/our-insights (Accessed: October 2024).
- 21. EY Global (2021) AI-Driven Auditing for Government Transparency. Available at: https://www.ey.com/en_gl/government-public-sector (Accessed: October 2024).