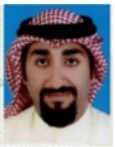
Dr. Meshari Abdulmajid Al-Ebrahim,
With the rising expectations for transparency and efficiency in the public sector, Artificial Intelligence (AI) and Machine Learning (ML) have become pivotal technologies in modern auditing practices. By automating routine tasks, improving data analysis, and enhancing risk assessment, AI and ML can help auditors become more efficient, effective, and accurate in their work. This article explores the historical context and the integration of AI and ML into public sector auditing, highlighting real-world applications and the implications for improving efficiency, accuracy, and decision-making. AI and ML offer significant opportunities, such as improved fraud detection and real-time monitoring. However, they also present challenges like data quality issues and cultural resistance. Additionally, this article examines the role of the Asian Organization of Supreme Audit Institutions (ASOSAI) in promoting the adoption of AI and ML. Leveraging his expertise, the author addresses these challenges and harnesses the full potential of these technologies to ensure the effective use of public resources. This article seeks to offer a thorough examination of the present state, potential future trends, and the essential equilibrium between innovation and ethical considerations in public sector auditing.
IntroductionThe auditing profession has undergone substantial changes over the years, with technological advancements playing a crucial role. Traditional auditing methods often involved manual reviews and spot checks, which were time-consuming and prone to human error. The introduction of AI and ML has revolutionised these practices, enabling real-time data analysis, continuous monitoring, and predictive analytics.
Public sector auditingplays a crucial role inensuringtransparency andeffectivegovernance. Inanage characterized by rapid technological advancements, the public sector is undergoing significant transformation. Among these changes, the integration of AI and ML into auditing processes stands out as a critical development. As governmental organisations strive to enhance their operational efficiency, AI and ML offer significant opportunities as well as considerable challenges in auditing.
The potential for these technologies to enhance the efficiency and effectiveness of audits has garnered attention from governments and auditing organisations worldwide. AI refers to the ability of machines to perform tasks that typically require human intelligence, such as understanding natural language, recognizing patterns, and making decisions. ML, a subset of AI, focuses on algorithms that enable systems to learn from data and improve over time. Therefore, AI and ML, by definition, refer to the development of intelligent systems capable of learning, reasoning, problem-solving, and perception
In the context of auditing, these technologies can be leveraged to enhance efficiency, accuracy, and effectiveness. By automating routine tasks, analysing vast datasets, and identifying patterns and anomalies, AI and ML can empower auditors to focus on higher-value activities, uncover hidden risks, and provide more insightful recommendations. The development of AI and ML technologies has progressed significantly in recent years, driven by advances in computational power and the availability of large datasets.
This article aims to explore how AI and ML can revolutionise public sector auditing by leveraging innovative technologies to improve accuracy, transparency, and decision-making. It will also discuss the challenges associated with adopting these technologies, such as data privacy, ethical concerns, and the need for auditor training. Additionally, the role of ASOSAI in promoting the adoption of AI and ML among its member countries will be examined. Overall, this article assesses the opportunities and challenges of using AI and ML in public sector auditing, drawing on recent research and practical applications to underscore their implications for audit quality and governance.
Opportunities Presented by AI and ML in Public Sector AuditingThe incorporation of AI and ML into public sector auditing presents several promising opportunities. One of the most significant benefits is the enhancement of efficiency and effectiveness. By automating time-consuming tasks such as data extraction, classification, and analysis, AI and ML allow auditors to focus on strategic activities that require critical thinking. For instance, these technologies can extract data from various sources, identify anomalies in financial data, and generate audit reports, leading to significant reductions in audit cycle times and improved overall efficiency.
Moreover, AI and ML algorithms offer improved accuracy by analysing vast datasets with greater precision than human auditors. They can effectively identify patterns and discrepancies that may indicate fraud or inefficiency, thus reducing the risk of human error and enhancing the overall effectiveness of audits. This is complemented by real-time monitoring and continuous auditing practices, which facilitate immediate detection of anomalies or potential fraud, allowing for timely interventions.
In addition to improving efficiency and accuracy, AI and ML can ensure compliance with regulations and policies through continuous monitoring of transactions and processes. These technologies provide data-driven insights that inform decision-making, helping auditors prioritise their work and allocate resources more effectively. By analysing large datasets, AI and ML can uncover trends, correlations, and causal relationships that may not be immediately apparent, further improving audit quality.
Causal knowledge discovery is another critical opportunity presented by AI and ML. The author’s findings can help auditors understand the underlying factors contributing to financial discrepancies, ultimately enhancing audit quality. For instance, the methodology developed for predictive modeling in risk management could be adapted to public sector auditing, demonstrating how identifying causal relationships can lead to more accurate assessments and better decision-making.
Additionally, AI and ML revolutionize risk identification and assessment through predictive analytics. By analyzing historical data and identifying unusual patterns or inconsistencies, auditors can proactively detect potential risks and take preventative measures, such as identifying fraudulent activities in transaction data.
The technologies also contribute to improved transparency in the public sector by enhancing oversight and detecting irregularities, ensuring that public funds are used effectively and efficiently. AI-powered systems can produce detailed and informative reports that enhance the transparency of auditing processes, ultimately increasing public trust in government institutions. Furthermore, the automation of audit processes significantly reduces costs associated with manual audits. To summarize, AI and ML opportunities in public sector auditing can be concluded as:
- Automated Compliance.
- Causal Knowledge Discovery.
- Fraud and Anomaly Detection.
- Data-Driven Decision-Making.
- Predictive Analytics for Risk Management.
- Cost Reduction and Resource Optimization.
- Real-Time Monitoring and Continuous Auditing.
- Improved Accuracy, Efficiency and Transparency.
Despite the numerous benefits, the adoption of AI and ML in public-sector auditing presents several challenges. While these challenges are significant, several strategies and best practices can help mitigate these issues and enhance the adoption process. Below are the challenges and best practices for implementing AI and ML in auditing:
-
Data Quality and Integrity: The quality and availability of data are critical in ensuring the successful
implementation of AI and ML in auditing. Inaccurate or incomplete data can lead to misleading conclusions and
undermine the reliability of audit findings. Public sector auditors often face challenges in obtaining and cleaning
data.
- Best Practice: Establish strong data governance frameworks to guarantee the quality and accessibility of data. Implement data standards and practices to guarantee the accuracy, completeness, and consistency of data. Conduct regular audits of data systems and adopt advanced data-cleaning algorithms to maintain data integrity. The author's expertise in data analysis can help address these challenges. By applying his methodologies, auditors can ensure that data used in AI and ML systems is accurate and reliable.
-
DataPrivacy andSecurity Concerns:The integrationofAI raises concerns about the confidentiality andsecurityof
sensitive data.
- Best Practice: Handle sensitive data with robust security measures to protect against breaches. A hybrid approach that combines human expertise with AI-driven insights can maximise audit efficiency and effectiveness by allowing human auditors to interpret findings and apply contextual understanding to complex situations.
-
Complexity of AI Systems and Interpretability Issues: The intricacies of AI models can lead to difficulties in
understanding and interpreting results. Implementing and using AI and ML tools in auditing can be technically
challenging and require specialised skills and expertise.
- Best Practice: Collaborate with technology providers and experts to facilitate the implementation and use ofAI and MLtools.Establishpartnerships witheducational institutions andtechcompanies for specialisedtrainingto bridge the knowledge gap.
-
Integration Issues: Integrating AI and ML solutions with existing auditing systems can be a technical challenge.
Organizations may face difficulties harmonizing new technologies with legacy systems, requiring investment in
both time and resources.
- BestPractice:Explore cost-effective solutions forAI and MLimplementation, suchas cloud-basedservices and open-source tools. Provide training for auditors to ensure a smooth integration process.
-
Legal andEthical Considerations:The implementationof AI and MLbrings forth important legal andethical issues,
especially concerning data privacy and protection.
- Best Practice: Develop and adhere to clear ethical guidelines for the responsible use of AI and ML in public sector auditing. Establish frameworks for data privacy, security, and the fair use of algorithms. Collaborate with regulatory bodies to ensure compliance with national and international laws governing AI use.
-
Cultural Resistance: The introduction of AI and ML may meet resistance from auditing professionals who fear that
automation could replace their roles.
- Best Practice: Implement effective change management strategies to overcome cultural resistance. Emphasize the role of technology as an augmentation of human capabilities rather than a replacement. Provide continuous training programs for audit professionals, focusing on the technical aspects of AI and ML and their application in auditing processes.
By addressing these challenges and adopting best practices, public sector organizations can effectively leverage AI and ML technologies to enhance audit quality and governance.
Current Applications for AI and ML in Public Sector AuditingThe application of AI and ML in public-sector auditing is gaining momentum globally. The following table summarizes real-world use cases, highlighting how various countries have implemented these technologies to improve their auditing practices.
To illustrate the potential of AI and ML in public sector auditing, it is beneficial to examine real-world case studies from various fields, including manufacturing engineering. One notable example is the author's research on causal knowledge discovery in manufacturing processes using a modified random forest-based predictive model. This study demonstrates how AI and ML can identify causal relationships between variables, which can be applied in auditing to uncover the root causes of anomalies or risks. Furthermore, the author's work on predictive modelling can help identify high-risk areas within financial statements, enabling auditors to allocate resources more effectively. Among the key benefits of these technologies is improved accuracy. The findings from this research can be appliedtopredict financial risks andanomalies inauditing, thereby enhancingboththe accuracy andefficiencyof audits. This capability not only assists auditors in identifying discrepancies but also streamlines the overall auditing process.
By studying such case studies, Supreme Audit Institutions (SAIs) can learn from the experiences of others and identify best practices for implementing AI and ML in their organizations.
AI and ML in the Context of Sustainable Development Goals (SDGs)AI and ML play a critical role in contributing to the achievement of several SDGs by enhancing public sector governance and efficiency. AI can be used to improve access to education and healthcare, reduce poverty, and promote sustainable cities and communities. Meanwhile, ML can enhance agricultural productivity, protect the environment, and promote gender equality. AI and ML align with specific SDGs in the following ways:
- SDG 8 (Decent Work and Economic Growth): ML models optimize the allocation and use of public funds, leading to more efficient government spending that supports economic growth. By reducing waste and increasing transparency, AI helps create environments that foster economic stability and opportunities for decent work. By optimizing resource allocation and reducing fraud, AI and ML contribute to sustainable economic growth.
- SDG 9 (Industry, Innovation, and Infrastructure): The use of AI and ML in public sector audits fosters innovation withingovernment institutions, encouragingthe adoptionof modern technologies.This innovation leads to more efficient public sector infrastructures and streamlined auditing processes, supporting overall societal development.
- SDG 11 (Sustainable Cities and Communities): AI-driven audits contribute to the sustainable management of public resources in urban planning, ensuring that funds allocated for infrastructure projects are used effectively and transparently. AI’s ability to monitor large datasets related to city planning helps governments create smarter, more resilient communities.
- SDG 16 (Peace, Justice, and Strong Institutions): AI and ML help build effective and transparent government institutions by improving the accuracy and efficiency of audit processes. Fraud detection, real-time monitoring, anddata-drivendecision-makingreduce corruption andenhance thegovernanceofpublic funds, contributingto the goals of building strong and transparent institutions.
The Asian Organization of Supreme Audit Institutions (ASOSAI), as a regional group ofINTOSAI, plays a crucial role in promoting the use of AI and ML in public sector auditing to enhance transparency and effectiveness. ASOSAI actively encourages its member institutions to adopt these technologies and provides training, resources, and platforms for knowledge sharing.This sectionoutlines how ASOSAI can leadinitiatives andpromote the adoptionof these technologies within its member countries.
ASOSAI can facilitate knowledge sharing through workshops, seminars, and collaborative projects focused on the integration of AI and ML in auditing practices. By encouraging cross-border knowledge exchange, ASOSAI can help audit institutions across Asia adopt best practices in AI-driven auditing. Additionally, ASOSAI can play a key role in setting ethical standards for the use of AI and ML in auditing within the Asian region. By issuing guidelines that address transparency, data privacy, and algorithmic fairness, ASOSAI can ensure that public sector audits remain ethical and aligned with regional values.
Advocating for policies and regulations that support the use of AI and ML in auditing is another critical role for ASOSAI. This involves promoting the development and implementation of frameworks that facilitate the integration of these technologies. To promote the innovative use of AI and ML, ASOSAI can sponsor research initiatives and encourage member organizations to explore new applications of these technologies in auditing. This fosters a culture of innovation and contributes to the development of more sophisticated audit techniques across the region.
As part ofits commitment to supporting the UN SDGs, ASOSAI can promote the use of AI and ML in audits related to SDG projects, particularly in areas like public finance management, environmental auditing, and healthcare expenditure audits. ASOSAI’s efforts highlight their commitment to leveragingtechnology to improvepublic sector auditing practices and align with the SDGs.
Future Perspectives and Recommendations in AI and MLThe future of public sector auditing lies in the continuous evolution of AI and ML technologies. Governments and audit institutions must remain adaptive, continuously upgrading their technological infrastructure and developing new auditing methodologies. Key areas for future research and development include exploring emerging trends and innovations in AI and ML for auditing, such as advanced predictive analytics and automated audit report generation.
Explainable AI focuses on creating AI models that offer transparent and comprehensible justifications for their decisions. Meanwhile,federated learning allows AI models to be trained on distributed data while ensuring privacy is maintained. As deep learning models become more advanced, they could be used for predictive auditing, where algorithms forecast potential risks or areas of non-compliance before they occur. This proactive approach marks a significant shift from traditional reactive auditing methods.
Increased automation is another trend as AI technologies mature, leading to the automation of repetitive tasks. This allows auditors to focus on higher-order analytical functions that demand human judgment and expertise. The development of collaborative AI systems that work alongside human auditors will enhance audit processes, combining the strengths of both technology and human insight for improved outcomes.
In the future, AI-assisted decision-making could extend beyond detecting anomalies to become integral to decision-making processes. AI could provide recommendations based on predictive analysis, helping auditors make informed, data-backed decisions in real-time. Additionally, AI-driven policy audits could assess the effectiveness of public policies. By analyzing outcomes and correlating them with policy inputs, AI could offer insights into the efficacy of government programs and recommend policy improvements.
As AI and ML become integral to auditing practices, comprehensive regulatory frameworks will be necessary to address ethical considerations and protect data privacy. Policymakers must work closely with auditing bodies to establish guidelines that promote responsible AI use. This includes developing standards for ethical AI usage in auditing, as well as ensuring data quality and governance.
Anticipating potential innovations and advancements in AI and ML technologies can further enhance auditing practices, including shifts toward more predictive and proactive auditing approaches. Investing in training and development is crucial to equip auditors with the skills and knowledge needed to effectively use AI and ML. Building AI and ML competencies within SAIs and promoting knowledge sharing and collaboration are essential steps.
ASOSAI plays a significant role in promoting the adoption of AI and ML in auditing among its member institutions. It fosters collaboration and knowledge sharing, advocating for policies and regulations that support the use of these technologies. By sponsoring research initiatives and encouraging innovative applications of AI and ML, ASOSAI contributes to the development of more sophisticated audit techniques across the region.
The COVID-19 pandemic has significantly accelerated the adoption of AI and ML technologies in the auditing sector. As organizations faced unprecedented challenges—such as remote work, disruptions in operations, and heightened regulatory scrutiny—the need for more efficient and effective auditing processes became paramount. The pandemic has acted as a catalyst for the integration of AI and ML in auditing, driving innovation and reshaping the future of the profession. This ongoing evolution of these technologies is likely to lead to more efficient, accurate, and insightful auditing practices in the years to come.
ConclusionThe integration of AI and ML into public sector auditing offers transformative potential, significantly enhancing efficiency, accuracy, and decision-making. These technologies symbolize the future of public sector governance, improving operational effectiveness and fostering transparency while aligning with the UN's SDGs. However, realizing their full potential requires addressing challenges related to data quality, cultural resistance, and ethical considerations. Overcoming these challenges will necessitate a collaborative effort across public institutions, facilitated by organizations such as ASOSAI.
By leveraging the insights of experts and embracing a forward-thinking approach, public sector auditors can navigate this evolving landscape. Harnessing the full potential of AI and ML will contribute to stronger, more transparentgovernance for all. Indoingso, these technologies will ensure the effective useofpublic resources,foster greater trust in government operations, and ultimately enhance the quality of life for citizens.
References- 1) Li, Y., & Goel, S. (2024). "Making It Possible for the Auditing of AI: A Systematic Review of AI Audits and AI Auditability." Information Systems Frontiers, Springer.
- 2) Mökander, J. (2023). "Auditing of AI: Legal, Ethical and Technical Approaches." Digital Society, 2(49), Springer.
- 3) Leocádio, D., Malheiro, L., & Reis, J. (2024). "Artificial Intelligence in Auditing: A Conceptual Framework for Auditing Practices." Administrative Sciences, 14(10), 238, MDPI.
- 4) Fedyk, A., Hodson, J., Khimich, N., & Fedyk, T. (2022). "Is artificial intelligence improving the audit process?" Review of Accounting Studies, 27(3), 938-9851.
- 5) Beckstrom, J. R. (2021). "Auditing Machine Learning Algorithms: A White Paper for Public Auditors." INTOSAI Journal.
- 6) Afsay, A., et al. (2023). "Auditing and Testing AI – A Holistic Framework." Journal of Accountancy.
- 7) Raj, S., & Seamans, R. (2022). "Artificial Intelligence and the Future of the Internal Audit Function." Journal of Internal Auditing.
- 8) Wolters Kluwer (2024). "Artificial intelligence in auditing: Enhancing the audit lifecycle."
- 9) IRS. (2020). “Use of Machine Learning for Tax Fraud Detection”.
- 10) NHS. (2018). “NLP in Healthcare Claims Audit”.
- 11) Australian Department of Human Services. (2019). “AI for Welfare Payments Monitoring”.
- 12) Canada Revenue Agency. (2021). “Machine Learning in Tax Evasion Identification”.
- 13) Comptroller and Auditor General of India. (2019). “Government Expenditure Audit with ML”.
- 14) South Korean Ministry of Economy and Finance. (2018). “Public Spending Monitoring with AI”.
- 15) European Court of Auditors. (2017). “Financial Transactions Analysis Using ML”.
- 16) Hai, T., Asadollahzadeh, M., Chauhan, B. S., Al-Ebrahim, M. A., Bunian, S., Eskandarzade, A., Salah, B. (2024). “Machine learning optimization and 4E analysis of a CCHP system integrated into a greenhouse system for carbon dioxide capturing”, Energy, Volume 309, 133028, ISSN 0360-5442. doi.org/10.1016/j.energy.2024.133028.
- 17) Bunian, S., Al-Ebrahim, M. A., & Nour, A. A. (2024). “Role and Applications of Artificial Intelligence and Machine Learning in Manufacturing Engineering: A Review.”, Engineered Science. doi:10.30919/es1044
- 18) Al-Ebrahim, M. A., Bunian, S., & Nour, A. A. (2023). “Recent machine-learning-driven developments in e-commerce: Current challenges and future perspectives.”, Engineered Science. doi:10.30919/es1044
- 19) Milyani, A. H., Al-Ebrahim, M. A., Attar, E. T., Abu-Hamdeh, N. H., Mostafa, M. E., Nusier, O. K., … Le, B. N. (2023). “Artificial Intelligence Optimization and experimental procedure for the effect of silicon dioxide particle size in silicon dioxide/deionized water nanofluid: Preparation, stability measurement and estimate the thermal conductivity of produced mixture.”, Journal of Materials Research and Technology, 26, 2575–2586. doi:10.1016/j.jmrt.2023.08.074
- 20) Al-Ebrahim, M. (2020). "Manufacturing Process Causal Knowledge Discovery using a Modified Random Forest-based Predictive Model.", Swansea University