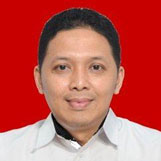
Mr. Mohammad Agus Prihandono,
Information Technology Bureau
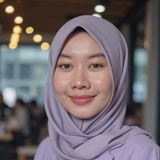
Ms. Candra Hapsari Susilo,
Finance and Accounting Department
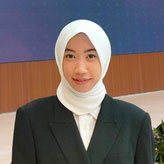
Ms. Reza Duratin Nasira,
Auditor
Introduction
In today’s world, nearly every aspect of life is intertwined with technology, driving rapid and transformative change. This transformation has led to the digitising of accounting records, rendering traditional books and documents obsolete (Usul & Alpay, 2024). Traditional document-centric review processes have evolved to data-driven approaches, where virtual environments generate and store data in integrated modules.
Furthermore, the Central Bank of The Republic of Indonesia highlights an increase in electronic money transactions, which soared from IDR 519.2 billion in 2009 to an impressive IDR 1,177.8 trillion by 2022 (Astari, et al., 2024). “This significant growth illustrates the rapid advancement and widespread adoption of electronic payment systems across the nation — showing an undeniable shift in how technology has affected lives.
With that in mind, the future of auditing heavily relies on technology, leveraging digital tools and data analytics to improve accuracy, efficiency, and insight throughout the auditing process. The approach will help to evaluate the accuracy and reliability of information systems without reliance on physical documentation (Doekhi, 2023). Audit firms are already testing and exploring the power of machine learning. Argus, a machine learning tool from Deloitte, can read documents such as leases, derivatives contracts, and sales contracts, effectively streamlining document review (Dickey, et al., 2019). However, adoptions of new technologies occasionally pose difficulties. Limitations in accessibility, infrastructure, and limited expertise are among the emerging challenges in adopting new technology (Jackson & Allen, 2024). In light of these developments, supreme audit institutions must also stay proactive, embracing technological innovation to meet the demands of an increasingly digital financial landscape, or risk falling behind as data complexity grows, yet still consider the challenges and potential hurdles in adopting new technologies.
Audits and Machine Learning: Opportunities and Challenges
Both private and public sectors have adapted quickly to the ever-changing world by digitising business processes, with surveys showing the majority of organizations see innovation as their top priority (Veltkamp & Jagesar, 2021). Following that, the variability of data to analyse during audits has increased. Traditional methods of audit risk identification were shown to be inadequate in overcoming the complexities and scope of modern transactions, reports, and datasets (Tian, et al., 2024). Addressing this issue, the incorporation of machine learning is a promising answer to audit risk assessment, ultimately ensuring the quality of assurance and accountability needed to make recommendations (Zuo, 2024)
To begin, the application of machine learning should be understood from the perspective of the technology acceptance model (TAM) proposed by Davis (1989). TAM determines whether a new technology should be adapted based on its benefits, enhanced output, and potential risks (Taib, et al., 2022). The implementation of new technologies according to TAM is decided by two factors, its perceived usefulness and perceived ease of use.
Adapted from Davis (1989)
Perceived Usefulness (PU) represents how valuable auditors believe machine learning can be in enhancing audit tasks, improving quality, and reducing errors. Perceived Ease of Use (PEOU), on the other hand, reflects auditors' perceptions of how simple and intuitive machine learning tools are to learn and incorporate into their workflow. Together, PU and PEOU shape auditors' attitudes toward Use, influencing their overall outlook on using machine learning. This positive attitude, in turn, drives Behavioral Intention to Use, which is the motivation to adopt and integrate machine learning tools into audit practices. Ultimately, the goal of TAM is Actual System Use—in this context, encouraging auditors to regularly use machine learning regularly based on its perceived benefits and ease of application.
Numerous studies have confirmed the significant role of machine learning in enhancing the effectiveness of audits. The use of machine learning algorithms enables auditors to examine and detect anomalies from large datasets efficiently and thoroughly quicker (Tan, 2023). Additionally, Peng & Tian (2023) identified a fraud detection strategy using a machine learning-based algorithm by recognizing diverging patterns in financial accounts. Conversely, Murugan & Kala (2023) suggested the use of a network analysis model to identify connections between steps in the business process, resulting in a more comprehensive detection of risk factors as well as vulnerabilities. Additionally, Hong, et. al. (2022) developed a text mining methodology by combining machine learning algorithms and natural language processing to skim through textual data in financial reports, audit documentation, and regulations which translated into insights. These approaches not only improve the auditors’ ability to detect risks but also to prevent and mitigate them. The use of machine learning allows for a more thorough examination of the expanding datasets, ultimately increasing the quality of assurance. From detection to insights, machine learning should conclusively be perceived as useful Various models have been shown to have had practical uses in audits. However, in terms of ease of use, further discussion still needs to be had. The application of a model would start from its development, from data preprocessing, and model creation to suit the business process of organizations, to the production phase and evaluation before translating into actual system use (Kang, 2024). While studies affirm the usefulness of machine learning, scepticism still lies in the accessibility, timeliness, and understanding of non-IT auditors in utilizing the machine learning model (Al Najjar, et al., 2024). We address these challenges in later sections.
Indonesia’s Digital Environment
With digital transformation being a major part of Indonesia’s national priority, digitalization has been a top priority in Indonesia, with digital transformation being a major part of Indonesia’s national priority for four years in a row in Indonesia’s Government Work Plan put together by The Ministry of National Development and Planning (2023) and sanctioned by the President of Indonesia. Based on Indonesia’s Government Work Plan, the goal is to not only strengthen the nation’s digital infrastructure, but also the digitalization of data regarding various fields including finance, agriculture, tourism, manufacturing, and the development of e-government.
Furthermore, in 2024, Indonesia’s E-Government Development Index (EGDI) reached a score of 0,79, ranked 64 out of 193 countries (United Nations, 2024). Following Indonesia’s digital roadmap formulated by the Ministry of Communication and Technology (2022), this number is set to increase in 2025. Given these circumstances, there will be significant changes in how audit documents are managed. The anticipated rise in digital evidence urges a proactive adaptation to ensure that recommendations and opinions are accurate, enhancing accountability across sectors. As a response to this shift, machine learning emerges as a powerful solution, offering the potential to transform auditing processes and strengthen transparency at every level.
Our Progress: BIDICS
Adapting to the current issue of digitalization in Indonesia, SAI of Indonesia has developed the BIDICS-BPK (Big Data Analytics) model. As a supreme audit institution, access to various data across various fields in the government is a given. BIDICS enables auditors to leverage algorithm-based statistical models to conduct broader data analysis than previously possible.
BIDICS can provide insights, oversights, as well as foresight depending on the request made by the users. Its development enables rapid identification of anomalies within large datasets, providing value through descriptive, diagnostic, and prescriptive analytics.
Gather, Detect, Analyze, and Assist
The implementation of BIDICS for audits mainly focuses on two processes, exploratory, and confirmatory. In the exploratory step, data are processed which then produces output that will show irregularities, anomalies, or certain events. The output will then be further confirmed by auditors to the related parties.
Grand Design of BIDICS-BPK (2021)
Data source refers to a set of data and information required to process that data. The BIDICS model uses two data sources, namely internal and external. Internal data is obtained from the ready application data. Ready application data refers to input data collected by the auditor in the field or received from the entity. External data is obtained from the national procurement application (LPSE), which includes procurement data, technical specifications, and budget amounts. The data from both sources consists of structured and unstructured data, which can be used as a model for analytic machine analysis. The machine learning technique analyses and matches the data obtained from both external and internal data, then present it with a visualization model.
Data and input are processed during the exploratory steps. The BIDICS model can analyse both structured and unstructured data. Structured data typically consists of clear rows, columns, and defined limits. Unstructured data, are those without a predefined form and exist in various formats, including images, text, voices, or videos. Structured data will then be batch processed while unstructured data will be stream processed in the analytic machine. The result of the analysis will then be stored in the repository. Auditors can access the result from the dashboard, in the form of visualized data or API that can be processed by other data processing applications such as Excel.
Visualization is a data dashboard that displays visual representations to present various types of processed data with analytic data, all placed in a centralised display. This display combines data from both internal and external data sources. Visualization modelling helps auditors and decision-makers to read and analyse potential events that may occur within an entity, enabling auditors and decision-makers to assess risks that need to be further explored during subsequent investigations.
Much like research, the initiation of BIDICS begins with a question. BIDICS focuses on a goal-oriented output. The process starts when a request is made by auditors, which then will be forwarded to IT teams. Then, both parties will discuss which data are readily available to be processed, and whether further data needs to be provided. Once acquired, analytics will begin. Outputs can come in the form of descriptive analytics, diagnostic analytics, discovery analytics, and predictive analytics. Any data and output will then be stored in the repository for future use.
Navigating Challenges
As previously mentioned, the TAM framework proposes two primary factors in the adoption of new technology; the perceived usefulness, and its perceived ease of use. Based on previous research, the use of machine learning in audits generally has a good perceived usefulness, however, the results of ease of use vary between the tech-savvy and your run-of-the-mill auditors. Challenges still lie in whether or not the user has a basic understanding of data processing. Not to mention certain specifications need to be met for devices to process large quantities of data (Al Najjar, et al., 2024).
For BIDICS, the perceived usefulness is legalized in the General Secretary of the Audit Board of Indonesia Decree No. 206/K/X-XIII.2/8/2021 on the Grand Design of BIDICS. Beyond its essential functions in descriptive, diagnostic, and predictive analytics, BIDICS is poised to become a key component of a broader digital ecosystem within Indonesia’s Public Finance Analytics Centre, a major initiative underway by the SAI of Indonesia. This role will be crucial in advancing transparent, accountable governance across the public sector.
Additionally, to address the concerns regarding ease of use, BIDICS offers two methods for data processing. In the first, users can self-process data through the Interactive Portal Data, accessing existing repository data or uploading their own for processing and analysis directly on their devices. The second method allows users to request support from the BIDICS Laboratory, which includes a team of data analysts, engineers, and scientists who work collaboratively with auditors to deliver tailored analytics. This dual approach empowers auditors, regardless of their technical background, to fully utilize BIDICS and accelerates the integration of machine learning into auditing. With these resources at their disposal, auditors can become proficient with machine learning, enhancing both their effectiveness and the audit process overall. While further testing still needs to be done in other to prove the practicality of this model empirically, current features are a step in the right direction towards a positive impact on the behavioural intention of machine learning implementation for audits in the public sectors, especially in Indonesia.
Conclusion
Achieving widespread usability requires careful attention to both perceived usefulness and ease of use, as outlined in the TAM framework. BIDICS stands as a valuable tool in this endeavour , backed by formal recognition and designed with both flexibility and support systems to accommodate users with varying levels of technical expertise. By providing accessible methods for data processing through both self-service and lab-assisted options, BIDICS not only enhances the efficiency and accuracy of auditing but also promotes a gradual and meaningful integration of machine learning into the audit process. This strategic approach will be pivotal in fostering a data-driven, transparent, and accountable governance environment within Indonesia’s public sector.
The implementation of machine learning in audits offers significant opportunities yet also brings notable challenges. It’s essential to assess readiness across manpower, infrastructure, and software. As auditors, we’re familiar with the importance of "substance over form"—prioritizing the true nature of transactions over formal appearance. In the same way, adopting new technology must be driven by functionality and effectiveness, not by the pursuit of novelty alone. Our focus should be on impactful, efficient integration that truly elevates and transforms the audit process.
References
Al Najjar, M., Ghanem, M. G., Mahboub, R., & Nakhal, B. (2024). The Role of Artificial Intelligence in Eliminating Accounting Errors. Journal of Financial Risk Management, 17(8). doi:https://doi.org/10.3390/jrfm17080353
Astari, C., Wahyuni, F. P., Larasati, D., Nuranjani, D., & Fadilla, A. (2024). Pengaruh Penggunaan Transaksi Digital Terhadap Pertumbuhan Ekonomi Akibat Dari Pandemi Covid-19. Journal of Business and Halal Industry, 1-10. doi:https://doi.org/10.47134/jbhi.v1i3.224
Davis, F. D. (1989). Perceived usefulness, perceived ease of use, and user acceptance of information technology. MIS Quarterly, 13(3), 319-340. doi:https://doi.org/10.2307/249008
Dickey, G., Blanke, S., & Seaton, L. (2019). Machine Learning in Auditing. The CPA Journal, 89(6), 16-21. Dipetik November 1, 2024, dari https://www.cpajournal.com/2019/06/19/machine-learning-in-auditing/
Doekhi, R. (2023). The Intercompany Settlement Blockchain: Benefits, Risks, and Internal IT-Controls. Dalam E. Berghout, R. Fijneman, L. Hendriks, M. de Boer, & B.-J. Butjin (Penyunt.), Advanced Digital Auditing, Theory and Practice of Auditing Complex Information (hal. 47-87). Springer. doi:10.1007/978-3-031-11089-4_4
Hong, S., Wu, H., Xu, X., & Xiong, W. (2022). Early Warning of Enterprise Financial Risk Based on Decision Tree Algorithm. Computational Intelligence and Neuroscience, 2, 1-9. doi:10.1155/2022/9182099
Jackson, D., & Allen, C. (2024). Enablers, barriers and strategies for adopting new technology in accounting. International Journal of Accounting Information Systems, 52. doi:https://doi.org/10.1016/j.accinf.2023.100666
Kang, H. (2024). Optimization of Enterprise Accounting Audit Risk Identification and Prevention Strategy Based on Machine Learning. Journal of Electrical Systems, 20(9), 79-84. Diambil kembali dari https://journal.esrgroups.org/jes/article/view/4292
Murugan, M. S., & Kala T, S. (2023). Large-scale data-driven financial risk management & analysis using machine learning strategies. Measurement: Sensors, 27, 100756. doi:10.1016/j.measen.2023.100756
Peng, C., & Tian, G. (2023). Intelligent auditing techniques for enterprise finance. Journal of Intelligent Systems, 32(1). doi:10.1515/jisys-2023-0011
Taib, A., Awang, Y., Shuhidan, S. M., Rashid, N., & Hasan, M. S. (2022). Digitalization in Accounting: Technology Knowledge and Readiness of Future Accountants. Universal Journal of Accounting and Finance, 10(1), 348-357. doi:10.13189/ujaf.2022.100135.
Tan, X. (2023). Enterprise Information Internal Control Management Based on Machine Learning Algorithm. 2023 2nd International Conference on Data Analytics, Computing and Artificial Intelligence (ICDACAI), 546-550. doi:10.1109/ICDACAI59742.2023.00109
Technology, M. o. (2022). Digital Roadmap 2021-2024. Diambil kembali dari https://aptika.kominfo.go.id/wp-content/uploads/2022/06/Road-Map-Reformasi-Birokrasi-Kominfo-Tahun- 2020-2024-1.pdf
The Ministry of National Development and Planning of the Republic of Indonesia (BAPPENAS RI). (2023). Government Work Plan. Presidential Decree No. 52 Year 2024. The Republic of Indonesia. Diambil kembali dari https://jdih.bappenas.go.id/peraturan/detailperaturan/3361/peraturan-presiden-nomor-52-tahun-2023
Tian, X., Tian, Z., Khatib, S. F., & Wang, Y. (2024). Machine learning in internet financial risk management: A systematic literature review. PLOS One, 19(6). doi:https://doi.org/10.1371/journal.pone.0300195
United Nations. (2024). E-Government Development Index. Diambil kembali dari https://publicadministration.un.org/egovkb/Data-Center
Usul, H., & Alpay, M. (2024). From Traditional Auditing to Information Technology Auditing: A Paradigm Shift in Practices. European Journal of Digital Economy Research, 5(1), 3-9. doi:https://doi.org/10.5281/zenodo.12819118
Veltkamp, C., & Jagesar, W. (2021). The impact of technological advancement in the audit. KPMG. Compact. Diambil kembali dari https://www.compact.nl/pdf/C-2021-3-Veltkamp.pdf
Zuo, Y. (2024). Automatic Analysis System for Enterprise Financial Information Based on Artificial Intelligence. International Conference on Integrated Circuits and Communication Systems (ICICACS), 1-5. doi:10.1109/ICICACS60521.2024.10499135
1As part of the Indonesia’s Government Work Plan put together by The Ministry of National Development and Planning (2023) and sanctioned by the President of Indonesia.