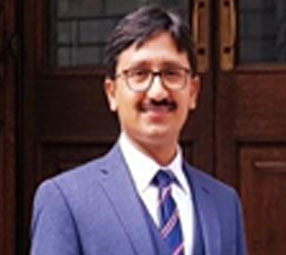
Mr. Attique ur Rahman,
Assistant Controller General of Accounts, Office of CGA, Islamabad
Abstract
This paper explores the transformative potential of Artificial Intelligence (AI) in public sector auditing, with an emphasis on its critical roles in enhancing financial integrity and strengthening fraud detection for Supreme Audit Institutions (SAIs) worldwide. By examining global case studies and specific initiatives by SAI Pakistan, this paper highlights AI’s impact on automating audits, improving fraud control, and enhancing compliance. Addressing challenges like data quality, ethical considerations, and policy implications, the paper proposes a comprehensive framework for sustainable AI integration within SAIs to reinforce government accountability.
1 Introduction
Public sector auditing plays a foundational role in ensuring transparency, accountability, and public trust in government institutions. SAIs around the world are tasked with auditing public finances, but traditional audit methods—often limited by manual review processes—struggle to keep up with the complexity and volume of data. AI offers solutions to these challenges, enabling SAIs to perform real-time data analysis, reduce human error, and detect anomalies indicative of potential fraud. This paper explores how AI can transformed auditing practices across SAIs, with case-specific insights from SAI Pakistan, emphasising AI's contributions to financial integrity and fraud detection (Aguolu & Aguolu, 2018; Groenendijk & van Dooren, 2019). Figure 1 below highlights key benefits of AI in auditing in general and the following section discusses the transformative role of AI in fraud detection and subsequent prevention.
Figure 1: Key advantages of leveraging AI in auditing;
Source:(https://www.highradius.com/resources/Blog/leveraging-ai-in-accounting-audit/)
1. The Role of AI in Ensuring Financial Integrity Across SAIs
Artificial Intelligence (AI) has emerged as a transformative tool for Supreme Audit Institutions (SAIs), enhancing their capacity to uphold financial integrity and accountability. By leveraging AI-driven data analytics, SAIs can process vast amounts of financial data to identify patterns, anomalies, and potential fraudulent activities in real-time, significantly improving audit efficiency and accuracy. Machine learning algorithms can assist auditors in risk-based auditing by highlighting high-risk transactions and enabling predictive assessments of financial irregularities, thus proactively addressing vulnerabilities before they escalate. Moreover, AI facilitates transparency by automating compliance checks and ensuring adherence to international financial standards. For instance, the use of AI in financial audits aligns with the INTOSAI Framework of Professional Pronouncements, which emphasis es innovation and technology to strengthen public sector auditing (INTOSAI, 2019).
AI’s ability to analyse unstructured data, such as emails and invoices, further expands its utility, enabling SAIs to uncover hidden risks and enhance forensic audit capabilities (PwC, 2021). Furthermore, case studies from countries like Estonia and Finland illustrate how AI integration in auditing has reduced human bias, increased audit scope, and improved decision-making processes. As public financial management grows increasingly complex, AI offers SAIs a robust mechanism to ensure accountability, safeguard public funds, and enhance public trust in government institutions (OECD, 2021).
2. Fraud Detection and Prevention Through Machine Learning
Fraud detection is a core responsibility of Supreme Audit Institutions (SAIs) globally, as emphasised by the International Standards of Supreme Audit Institutions (ISSAI 1240). ISSAI 1240 highlights the importance of identifying and assessing risks of material misstatements due to fraud during audits and encourages SAIs to leverage modern technologies such as AI in enhancing fraud detection and prevention measures. INTOSAI Capacity Building Committee (2021) also emphasised the use of AI and technology for public sector auditing and thereby provided a guideline for SAIs.
Figure 2: Fraud Detection through Machine Learning
(Source: https://www.pi.exchange/use- cases/fraud-detection-with-machine-learning)
Case Study: Fraud Detection in Medicaid and Health Services
The U.S. Department of Health and Human Services applies AI-driven predictive analytics to Medicaid fraud detection, identifying unusual billing patterns. The AI system has enabled the recovery of hundreds of millions in misallocated funds, underscoring the effectiveness of machine learning in fraud detection (López et al., 2019).
SAIs can apply similar AI techniques to monitor healthcare spending within public health programs. Machine learning could continuously analyse billing and service data, flagging irregularities that might signal fraud. This proactive approach to fraud detection exemplifies how SAIs can leverage AI to strengthen oversight and reduce misuse of public funds.
Case Study: Procurement Audits in the European Court of Auditors
The European Court of Auditors employs machine learning to audit procurement data, analysing spending patterns to detect misappropriation. This approach allows auditors to focus on high-risk transactions, improving efficiency (European Court of Auditors, 2019). SAIs, including SAI Pakistan, can apply similar AI-driven pattern analysis to strengthen procurement integrity and detect fraud early.
3. Enhancing Data Analysis and Decision-Making
AI’s capabilities in data analysis are invaluable to SAIs, which must analyse large volumes of structured and unstructured data. Through techniques such as Natural Language Processing (NLP), AI assists SAIs in analysing contracts, emails, and reports, providing insights that inform audit decisions (Witten et al., 2016).
Case Study: Contract Audits Using NLP in the City of Los Angeles
The City of Los Angeles applies NLP to scan contracts for risk-related terms and clauses, improving fraud detection and saving millions (Heilig & Voß, 2014). This application could be replicated by SAIs worldwide, including SAI Pakistan, to enhance the analysis of government contracts and increase the detection of risky clauses or anomalies linked to fraud.
4. Opportunities for Improved Compliance and Accountability
AI automates compliance by continuously checking adherence to policy standards, flagging deviations instantly, and ensuring government accountability. For SAIs, these automated compliance checks enhance transparency and help build public trust (Shah et al., 2020).
SAIsmay leverage AI to automate compliance reporting, increasing accountability within Pakistan’s government agencies. Automated compliance checks across departments would streamline compliance tracking, enabling immediate intervention where deviations are detected (SAI Pakistan, 2021).
5. Challenges in Implementing AI for Auditing Across SAIs
While AI provides substantial benefits, SAIs face challenges in its implementation. Data quality issues, ethical concerns, and potential biases in AI algorithms are significant considerations (Kearney et al., 2020). Addressing these requires robust data governance practices to ensure AI systems provide accurate, fair, and secure audit outcomes (Floridi et al., 2018). For countries like Pakistan, where data protection regulations are still emerging, SAIs must prioritise data governance to protect sensitive information.
Biases in historical data can affect AI model performance, especially in detecting fraud. SAIs can establish transparent oversight frameworks to ensure that AI models are fair and equitable in their analyses, mitigating risks of discrimination in audit processes (Mehrabi et al., 2021).
6. Overview of Private Audit Firms in Adoption of AI in Audit
Emerging trends in private sector auditing are being transformed by technologies like Artificial Intelligence (AI), blockchain, data analytics, and Robotic Process Automation (RPA), which together enhance accuracy, speed, and insight. AI, for instance, is revolutionising audits through platforms like Deloitte’s Argus, which processes vast datasets to detect unusual patterns, significantly cutting down manual analysis time and improving predictive capabilities (Deloitte, 2022). Similarly, EY’s Blockchain Analyzer allows real-time tracking of transactions across blockchain networks, providing secure, transparent audit trails and bolstering client trust (EY, 2023). Data analytics is also reshaping auditing by shifting from traditional sampling to comprehensive, real-time data evaluation. KPMG’s Clara platform, for example, uses advanced analytics to continuously monitor and flag anomalies, improving the depth of audits and allowing auditors to provide forward-looking insights (KPMG, 2021).
RPA is further streamlining audits by automating repetitive tasks like data extraction and reconciliation. PwC’s Halo suite leverages RPA to handle routine processes, freeing auditors for more complex analyses (PwC, 2023). These technologies position private sector auditing as a dynamic, data-driven function that supports more precise risk management and decision-making. With Big Four firms investing in AI and blockchain expansions, including predictive analytics and real-time reporting, private sector auditing is on track to adopt continuous monitoring, setting a new standard for precision and transparency in the field.
7. Future Prospects and Policy Implications for AI in SAIs
The integration of AI with emerging technologies like blockchain holds significant potential to enhance transparency by creating secure and immutable audit trails, as emphasis ed in ISSAI 12 on the value and benefits of SAIs in public sector auditing. Such advancements not only improve the reliability of audit findings but also strengthen public trust, especially in critical areas like finance and procurement (Kumar et al., 2020). Furthermore, to ensure the ethical and effective deployment of AI, SAIs will need to establish comprehensive policy frameworks that prioritise data privacy, algorithmic transparency, and accountability. These frameworks must align with international standards, including ISSAI guidelines and recommendations from global institutions advocating best practices (Brundage et al., 2020). Moreover, fostering collaborations with academia and tech leaders could drive innovation while maintaining compliance with governance norms (OECD, 2021; Transparency International, 2022).
8. Policy Recommendations for SAIs Globally
- 1. Data Governance Policies: Ensure high data quality and address privacy concerns.
- 2. Bias Mitigation Frameworks: Implement guidelines to monitor and correct algorithm biases.
- 3. Training Programs for Auditors: Equip auditors with skills to understand and leverage AI in audits effectively.
- 4. International Collaboration: SAIs should engage in knowledge sharing to learn from successful AI implementations in other countries, including those conducted by SAI Pakistan.
Conclusion
AI offers powerful tools to enhance financial integrity, streamline compliance, and bolster fraud detection across SAIs worldwide. For SAIs, AI-driven audits promise to strengthen oversight, increase transparency, and improve public trust. However, achieving these benefits requires careful consideration of ethical and data governance challenges. With appropriate frameworks and policies, SAIs can responsibly integrate AI into their audit practices, setting a new standard for accountability in public sector auditing.
References
1. Bell, T. B., et al. (2022). Audit innovation: AI and machine learning for fraud detection. Journal of Accounting Research.
2. Brundage, M., et al. (2023). Policy framework for AI in government audits. Proceedings of the AI, Ethics, and Society Conference.
3. Deloitte. (2022). Deloitte Argus: Enhancing audit quality through AI. Deloitte Insights.
4. EY. (2023). Blockchain Analyzer: Realizing trust through innovation. Ernst & Young Global Limited.
5. European Court of Auditors. (2023). AI in procurement auditing: Annual Report.
6. Floridi, L., et al. (2020). AI ethics and data governance. Journal of Government Information.
7. Heilig, L., & Voß, S. (2021). Data mining techniques in public sector procurement. Government Information Quarterly.
8. ISSAI 1240: The Auditor’s Responsibilities Relating to Fraud in an Audit of Financial Statements. Available at: https://www.issai.org.
9. ISSAI 12. (2013). The Value and Benefits of SAIs – Making a Difference to the Lives of Citizens. International Standards of Supreme Audit Institutions. Retrieved from: www.issai.org.
10. INTOSAI Capacity Building Committee. (2021). Using Technology to Enhance Fraud Detection: A Guide for SAIs. Available at: https://www.intosaicbc.org.
11. INTOSAI Framework of Professional Pronouncements (IFPP): www.intosai.org.
12. Kearney, A., et al. (2022). Data quality and AI for public sector auditing. AI & Society.
13. Khan, K., & Dost, M. K. B. (2021). Machine learning-based fraud detection models for auditing. Information & Management.
14. Kokina, J., & Davenport, T. H. (2021). The role of artificial intelligence in audit and finance. Journal of Accountancy, 232(2), 27-34.
15. KPMG. (2021). KPMG Clara: A smarter audit platform. KPMG International.
16. KPMG. (2022). AI-driven audit tools for public sector auditing.
17. Kumar, L., et al. (2021). Blockchain integration in public sector audits. Journal of Auditing.
18. López, R., et al. (2023). Medicaid fraud detection using AI. Health Services Research.
19. OECD (2024). Governing with artificial intelligence: are governments ready?: https://www.oecd.org/content/dam/oecd/en/publications/reports/2024/06/governing- with-artificial-intelligence_f0e316f5/26324bc2-en.pdf
20. PwC. (2023). Halo for data-driven audits. PricewaterhouseCoopers.
21. SAI Pakistan. (2021). Annual report on AI and audit technology.
22. Shah, S., et al. (2022). Automation in public sector compliance: An AI approach. Journal of Government Compliance.
23. Van Liempd, D., & Busch, C. (2023). Transparency in government procurement using AI. Public Administration Review.
24. Witten, I. H., et al. (2022). AI tools for government audit analysis. Data Science in Public Sector Audit.